Kết hợp mô hình YOLOV4-TINY và LSTM để nâng cao độ chính xác của hệ thống cảnh báo người có hành vi hút thuốc lá nơi công cộng
Email:
linhvt_ph@utc.edu.vn
Từ khóa:
Hành vi hút thuốc; mạng nơ-ron tích chập; Deep learning; YOLOv4-tiny; Long Short Term Memory
Tóm tắt
Khói thuốc lá chứa nhiều chất hóa học, trong đó có nhiều chất gây ra những căn bệnh nguy hiểm điển hình như: ung thư phổi, bệnh tim mạch, vô sinh và nhiều bệnh nan y khác. Nhắc nhở và cảnh báo người hút thuốc lá nơi công cộng là một trong những việc làm quan trọng trong việc tuyên truyền và phòng chống tác hại của thuốc lá. Các hệ thống giám sát người hút thuốc lá và cảnh báo thông minh đã được quan tâm nghiên cứu bởi cộng đồng khoa học trong thời gian qua. Mục đích của nghiên cứu này là cải thiện hệ thống trước đây bằng cách sử dụng Yolov4 kết hợp LSTM để cho ra kết quả dự đoán chính xác cao. Thay vì chỉ sử dụng Yolov4 như là phương pháp phát hiện người cầm điếu thuốc lá, phương pháp đề xuất này sử dụng Yolov4 để trích các đặc trưng từ các khung hình của video, chuỗi các khung hình đặc trưng liên tiếp này được đưa vào mạng LSTM để dự đoán. Để đánh giá hiệu suất của phương pháp, nghiên cứu đã thực hiện trên bộ dữ liệu được thu thập riêng của nhóm: 5000 ảnh và 120 video chứa hành vi hút thuốc. Kết quả cho thấy rằng cách tiếp cận đề xuất đã thành công khi nhận ra các hành động hút thuốc lá của con người trên bộ dữ liệu này với độ chính xác cao hơn phương pháp truyền thốngTài liệu tham khảo
[1]. C. Fan, F. Gao, A New Approach for Smoking Event Detection Using a Variational Autoencoder and Neural Decision Forest, IEEE Access, 8 (2020) 120835-120849. https://doi.org/10.1109/ ACCESS.2020.3006163
[2]. A. L. Skinner, C. J. Stone, H. Doughty, M. R. Munafo, StopWatch: The Preliminary Evaluation of a Smartwatch-Based System for Passive Detection of Cigarette Smoking, Nicotine & Tobacco Research, 21 (2019) 257–261. https://doi.org/10.1093/ntr/nty008
[3]. Abhinav Parate, Meng-Chieh Chiu, Chaniel Chadowitz, Deepak Ganesan, Evangelos Kalogerakis, RisQ: recognizing smoking gestures with inertial sensors on a wristband. In Proceedings of the 12th annual international conference on Mobile systems, applications, and services, Association for Computing Machinery, New York, USA, (2014) 149–161. https://doi.org/10.1145/2594368.2594379
[4]. Chrisogonas O Odhiambo, Casey A Cole, Alaleh Torkjazi, Homayoun Valafar, State Transition Modeling of the Smoking Behavior Using LSTM Recurrent Neural Networks, International Conference on Computational Science and Computational Intelligence (CSCI), 2019, pp. 898-904. https://doi.org/10.1109/CSCI49370.2019.00171
[5]. S. W. Pienaar and R. Malekian, Human Activity Recognition using LSTM-RNN Deep Neural Network Architecture, IEEE 2nd Wireless Africa Conference (WAC), 2019, pp. 1-5. https://doi.org/10.1109/AFRICA.2019.8843403
[6]. Zhenkai Lin; Changfeng Lv; Yimin Dou; Jinping Li, Smoking Behavior Detection Based on Hand Trajectory Tracking and Mouth Saturation Changes, 2018 International Conference on Security, Pattern Analysis, and Cybernetics (SPAC), 2018, pp. 326-331. https://doi.org/10.1109/SPAC46244.2018.8965455
[7]. Tse-Lun Bien and Chang Hong Lin, Detection and recognition of indoor smoking events, Proc. SPIE 8784, Fifth International Conference on Machine Vision: Algorithms, Pattern Recognition, and Basic Technologies, 2013, 878424. https://doi.org/10.1117/12.2020967
[8]. Waynebert Jan D. Cabanto, Aira Danielle B. Jocson, Renzel Laurence T. Lateo, and Joel C. De Goma, Real-Time Multi-Person Smoking Event Detection, In Proceedings of the 2nd International Conference on Computing and Big Data (ICCBD 2019), Association for Computing Machinery, New York, NY, USA, 2019, pp.126–130. https://doi.org/10.1145/3366650.3366678
[9]. Võ Thiện Lĩnh, Triển khai hệ thống phát hiện và cảnh báo hút thuốc lá nơi công cộng dựa trên cấu trúc mạng YOLOv4-tiny, Hội nghị quốc gia lần thứ XXIV về điện tử, truyền thông và công nghệ thông tin REV – ECIT, 2021, pp. 182-186.
[10]. Xiangkui Jiang, Haochang Hu, Xun Liu, Rui Ding, Yuanbo Xu, Jianxu Shi, Yaoyao Du and Chunlin Da, A smoking behavior detection method based on the YOLOv5 network, Journal of Physics: Conference Series, 2021 International Conference on Control Theory and Applications, 2232 (2021) 012001. https://doi.org/10.1088/1742-6596/2232/1/012001
[11]. Almeida, Aitor, Gorka Azkune, Predicting Human Behaviour with Recurrent Neural Networks, Applied Sciences, 8 (2018) 305. https://doi.org/10.3390/app8020305
[12]. Joy Bhattacharjee, Soumen Santra, Arpan Deyasi, Chapter 10 - Novel detection of cancerous cells through an image segmentation approach using principal component analysis, Editor(s): Siddhartha Bhattacharyya, Paramartha Dutta, Debabrata Samanta, Anirban Mukherjee, Indrajit Pan, Recent Trends in Computational Intelligence Enabled Research, Academic Press, 2021, pp. 171-195. https://doi.org/10.1016/B978-0-12-822844-9.00035-9
[13]. Chandramouli Das, Abhaya Kumar Sahoo, Chittaranjan Pradhan, Chapter 12 - Multicriteria recommender system using different approaches, Editor(s): Sushruta Mishra, Hrudaya Kumar Tripathy, Pradeep Kumar Mallick, Arun Kumar Sangaiah, Gyoo-Soo Chae, In Cognitive Data Science in Sustainable Computing, Cognitive Big Data Intelligence with a Metaheuristic Approach, Academic Press, 2022, pp. 259-277. https://doi.org/10.1016/B978-0-323-85117-6.00011-X
[2]. A. L. Skinner, C. J. Stone, H. Doughty, M. R. Munafo, StopWatch: The Preliminary Evaluation of a Smartwatch-Based System for Passive Detection of Cigarette Smoking, Nicotine & Tobacco Research, 21 (2019) 257–261. https://doi.org/10.1093/ntr/nty008
[3]. Abhinav Parate, Meng-Chieh Chiu, Chaniel Chadowitz, Deepak Ganesan, Evangelos Kalogerakis, RisQ: recognizing smoking gestures with inertial sensors on a wristband. In Proceedings of the 12th annual international conference on Mobile systems, applications, and services, Association for Computing Machinery, New York, USA, (2014) 149–161. https://doi.org/10.1145/2594368.2594379
[4]. Chrisogonas O Odhiambo, Casey A Cole, Alaleh Torkjazi, Homayoun Valafar, State Transition Modeling of the Smoking Behavior Using LSTM Recurrent Neural Networks, International Conference on Computational Science and Computational Intelligence (CSCI), 2019, pp. 898-904. https://doi.org/10.1109/CSCI49370.2019.00171
[5]. S. W. Pienaar and R. Malekian, Human Activity Recognition using LSTM-RNN Deep Neural Network Architecture, IEEE 2nd Wireless Africa Conference (WAC), 2019, pp. 1-5. https://doi.org/10.1109/AFRICA.2019.8843403
[6]. Zhenkai Lin; Changfeng Lv; Yimin Dou; Jinping Li, Smoking Behavior Detection Based on Hand Trajectory Tracking and Mouth Saturation Changes, 2018 International Conference on Security, Pattern Analysis, and Cybernetics (SPAC), 2018, pp. 326-331. https://doi.org/10.1109/SPAC46244.2018.8965455
[7]. Tse-Lun Bien and Chang Hong Lin, Detection and recognition of indoor smoking events, Proc. SPIE 8784, Fifth International Conference on Machine Vision: Algorithms, Pattern Recognition, and Basic Technologies, 2013, 878424. https://doi.org/10.1117/12.2020967
[8]. Waynebert Jan D. Cabanto, Aira Danielle B. Jocson, Renzel Laurence T. Lateo, and Joel C. De Goma, Real-Time Multi-Person Smoking Event Detection, In Proceedings of the 2nd International Conference on Computing and Big Data (ICCBD 2019), Association for Computing Machinery, New York, NY, USA, 2019, pp.126–130. https://doi.org/10.1145/3366650.3366678
[9]. Võ Thiện Lĩnh, Triển khai hệ thống phát hiện và cảnh báo hút thuốc lá nơi công cộng dựa trên cấu trúc mạng YOLOv4-tiny, Hội nghị quốc gia lần thứ XXIV về điện tử, truyền thông và công nghệ thông tin REV – ECIT, 2021, pp. 182-186.
[10]. Xiangkui Jiang, Haochang Hu, Xun Liu, Rui Ding, Yuanbo Xu, Jianxu Shi, Yaoyao Du and Chunlin Da, A smoking behavior detection method based on the YOLOv5 network, Journal of Physics: Conference Series, 2021 International Conference on Control Theory and Applications, 2232 (2021) 012001. https://doi.org/10.1088/1742-6596/2232/1/012001
[11]. Almeida, Aitor, Gorka Azkune, Predicting Human Behaviour with Recurrent Neural Networks, Applied Sciences, 8 (2018) 305. https://doi.org/10.3390/app8020305
[12]. Joy Bhattacharjee, Soumen Santra, Arpan Deyasi, Chapter 10 - Novel detection of cancerous cells through an image segmentation approach using principal component analysis, Editor(s): Siddhartha Bhattacharyya, Paramartha Dutta, Debabrata Samanta, Anirban Mukherjee, Indrajit Pan, Recent Trends in Computational Intelligence Enabled Research, Academic Press, 2021, pp. 171-195. https://doi.org/10.1016/B978-0-12-822844-9.00035-9
[13]. Chandramouli Das, Abhaya Kumar Sahoo, Chittaranjan Pradhan, Chapter 12 - Multicriteria recommender system using different approaches, Editor(s): Sushruta Mishra, Hrudaya Kumar Tripathy, Pradeep Kumar Mallick, Arun Kumar Sangaiah, Gyoo-Soo Chae, In Cognitive Data Science in Sustainable Computing, Cognitive Big Data Intelligence with a Metaheuristic Approach, Academic Press, 2022, pp. 259-277. https://doi.org/10.1016/B978-0-323-85117-6.00011-X
Tải xuống
Chưa có dữ liệu thống kê
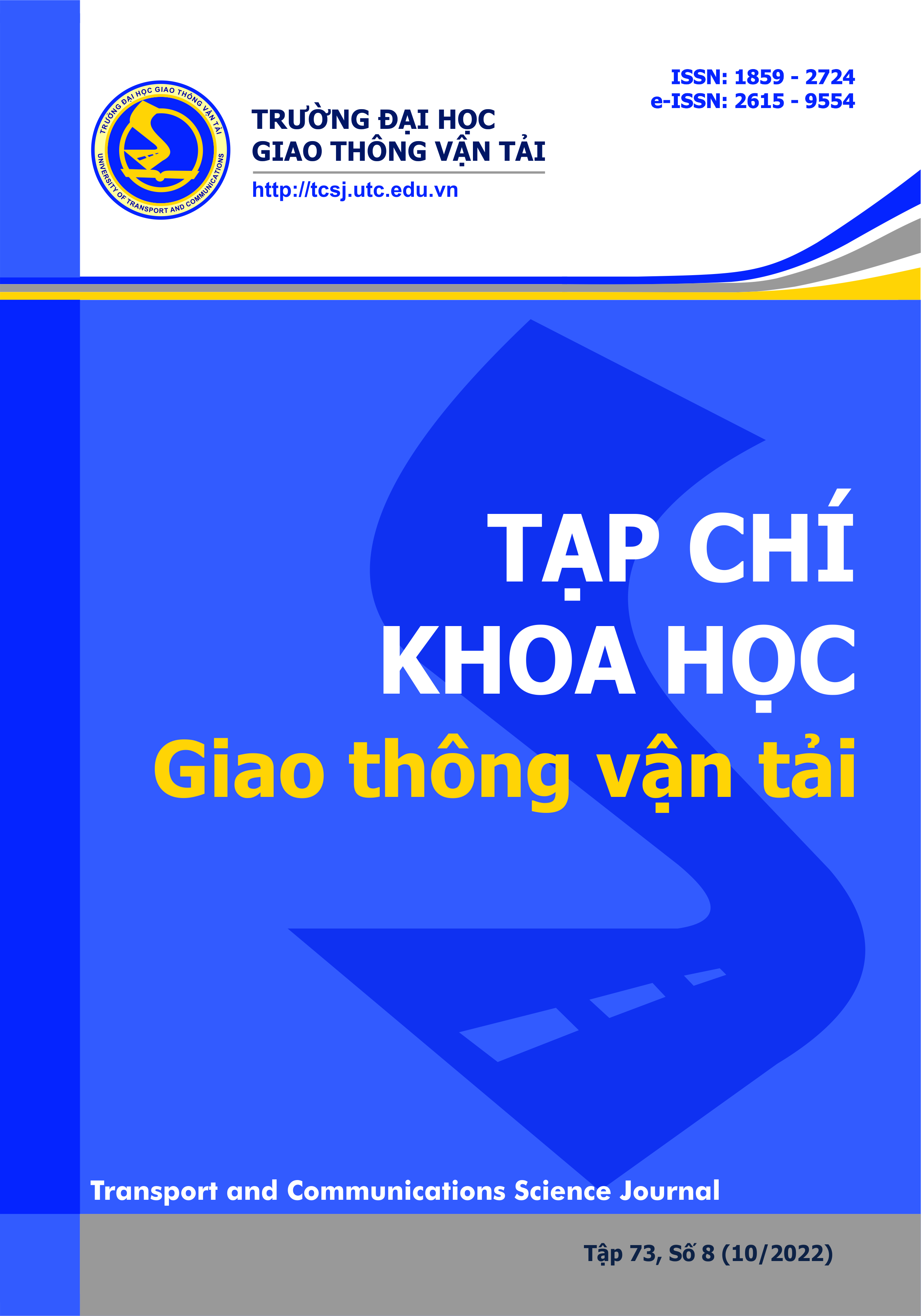
Nhận bài
24/08/2022
Nhận bài sửa
27/09/2022
Chấp nhận đăng
14/10/2022
Xuất bản
15/10/2022
Chuyên mục
Công trình khoa học
Kiểu trích dẫn
Võ Thiện, L. (1665766800). Kết hợp mô hình YOLOV4-TINY và LSTM để nâng cao độ chính xác của hệ thống cảnh báo người có hành vi hút thuốc lá nơi công cộng. Tạp Chí Khoa Học Giao Thông Vận Tải, 73(8), 785-797. https://doi.org/10.47869/tcsj.73.8.4
Số lần xem tóm tắt
267
Số lần xem bài báo
132