Nghiên cứu ứng dụng mô hình trí tuệ nhân tạo dự báo cường độ nén của bê tông sử dụng cốt liệu tái chế
Email:
banglh@utt.edu.vn
Từ khóa:
Cường độ nén, bê tông cốt liệu tái chế, mạng nơ ron nhân tạo (ANN), thuật toán Conjugate gradient.
Tóm tắt
Lĩnh vực xây dựng đang phát triển nhanh chóng trong thập kỷ qua. Các hoạt động này đòi hỏi một lượng lớn cốt liệu để xây dựng mới và cũng tạo ra một lượng lớn chất thải từ việc phá dỡ các công trình cũ. Để khắc phục điều này, bê tông cốt liệu tái chế (RAC), trong đó cốt liệu tự nhiên được thay thế bằng cốt liệu bê tông tái chế, hiện đang là một hướng nghiên cứu tiềm năng và giúp bảo tồn tài nguyên thiên nhiên, giảm thiểu tác động đến môi trường. Tuy nhiên, việc tối ưu hóa hỗn hợp RAC do thành phần khác nhau của cốt liệu tái chế và việc ước tính cường độ nén đòi hỏi các kỹ thuật mới và phức tạp. Trong nghiên cứu này, mô hình mạng nơ ron nhân tạo với thuật toán Conjugate gradient được đề xuất để dự đoán cường độ nén của RAC. Cơ sở dữ liệu RAC trong nghiên cứu này gồm 650 kết quả thí nghiệm được tổng hợp từ 69 nghiên cứu thử nghiệm. Hiệu suất của mô hình ANN được đánh giá bằng cách sử dụng các tiêu chí thống kê, cụ thể là hệ số tương quan (R), sai số toàn phương trung bình (RMSE), sai số tuyệt đối trung bình (MAE) và sai số phần trăm tuyệt đối trung bình (MAPE). Kết quả cho thấy mô hình ANN đề xuất là một công cụ dự đoán hợp lý và hữu ích cho các kỹ sư, giúp tiết kiệm thời gian, giảm thiểu các thí nghiệm tốn kém.Tài liệu tham khảo
[1] V. Radonjanin, M. Malešev, S. Marinković, A.E.S. Al Malty, Green recycled aggregate concrete, Construction and Building Materials, 47 (2013) 1503-1511. https://doi.org/10.1016/j.conbuildmat.2013.06.076
[2] O. Cakır, Experimental analysis of properties of recycled coarse aggregate (RCA) concrete with mineral additives, Construction and Building Materials, 68 (2014) 17-25. https://doi.org/10.1016/j.conbuildmat.2014.06.032
[3] F. Pacheco-Torgal, Y. Ding, S. Miraldo, Z. Abdollahnejad, J.A. Labrincha, Are geopolymers more suitable than Portland cement to produce high volume recycled aggregates HPC?, Construction and Building Materials, 36 (2012) 1048-1052. https://doi.org/10.1016/j.conbuildmat.2012.07.004
[4] M. Ghanbari, A.M. Abbasi, M. Ravanshadnia, Production of natural and recycled aggregates: the environmental impacts of energy consumption and CO2 emissions, Journal of Material Cycles and Waste Management, 20 (2018) 810–822. https://doi.org/10.1007/s10163-017-0640-2
[5] M. Bravo, J. De Brito, J. Pontes, L. Evangelista, Mechanical performance of concrete made with aggregates from construction and demolition waste recycling plants, Journal of Cleaner Production, 99 (2015) 59-74. https://doi.org/10.1016/j.jclepro.2015.03.012
[6] B. Gonzalez-Fonteboa, F. Martinez-Abella, Concretes with aggregates from demolition waste and silica fume, Materials and mechanical properties, Building and Environment, 43 (2008) 429-437. https://doi.org/10.1016/j.buildenv.2007.01.008
[7] I.B. Topcu, M. Sarıdemir, Prediction of mechanical properties of recycled aggregate concretes containing silica fume using artificial neural networks and fuzzy logic, Computational Materials Science, 42 (2008) 74-82. https://doi.org/10.1016/j.commatsci.2007.06.011
[8] Z.H. Duan, C.S. Poon, Properties of recycled aggregate concrete made with recycled aggregates with different amounts of old adhered mortars, Materials & Design, 58 (2014) 19-29. https://doi.org/10.1016/j.matdes.2014.01.044
[9] D. Pedro, J. De Brito, L. Evangelista, Performance of concrete made with aggregates recycled from precasting industry waste: influence of the crushing process, Materials and Structures, 48 (2015) 3965-3978. https://doi.org/10.1617/s11527-014-0456-7
[10] R.V. Silva, J. De Brito, R.K. Dhir, The influence of the use of recycled aggregates on the compressive strength of concrete: a review, European Journal of Environmental and Civil Engineering, 19 (2015) 825-849. https://doi.org/10.1080/19648189.2014.974831
[11] K. Naouaoui, A. Bouyahyaoui, T. Cherradi, Experimental Characterization of Recycled Aggregate Concrete, in: MATEC Web of Conferences, EDP Sciences, 303 (2019) 05004. https://doi.org/10.1051/matecconf/201930305004
[12] K. Rahal, Mechanical properties of concrete with recycled coarse aggregate, Building and Environment, 42 (2007) 407-415. https://doi.org/10.1016/j.buildenv.2005.07.033
[13] T.-Y. Tu, Y.-Y. Chen, C.-L. Hwang, Properties of HPC with recycled aggregates, Cement and Concrete Research, 36 (2006) 943-950. https://doi.org/10.1016/j.cemconres.2005.11.022
[14] Q.H. Nguyen, H.-B. Ly, V.Q. Tran, T.-A. Nguyen, V.-H. Phan, T.-T. Le, B.T. Pham, A novel hybrid model based on a feedforward neural network and one step secant algorithm for prediction of load-bearing capacity of rectangular concrete-filled steel tube columns, Molecules. 25 (2020) 3486. https://doi.org/10.3390/molecules25153486
[15] Lý Hải Bằng, Nguyễn Thùy Anh, Nghiên cứu dự báo sức chịu tải tới hạn của cấu kiện cột ống thép nhồi bê tông có tiết diện hình chữ nhật bằng mạng nơ ron nhân tạo, Tạp chí Khoa học Giao thông vận tải, 71 (2020) 154-166. https://doi.org/ 10.25073/tcsj.71.2.10
[16] Nguyễn Thùy Anh, Lý Hải Bằng, Dự đoán sức kháng cắt của dầm bê tông cốt thanh FRP không có cốt thép đai sử dụng mạng thần kinh nhân tạo, Tạp chí Khoa học Giao thông vận tải, 71 (2020) 1047-1060. https://doi.org/10.47869/tcsj.71.9.4
[17] Nguyễn Thùy Anh, Lý Hải Bằng, Phương pháp dự đoán tải trọng ổn định đàn hồi của dầm thép H bản bụng khoét lỗ tròn dựa trên máy vec-tơ hỗ trợ, Tạp chí Khoa học Giao thông vận tải, 71 (2020) 500-513. https://doi.org/10.25073/tcsj.71.5.4
[18] Nguyễn Thuỳ Anh, Lý Hải Bằng, Development of ANN-based models to predict the bond strength of GFRP bars and concrete beams, Tạp chí Khoa học Giao thông vận tải, 71 (2020) 814-827. https://doi.org/10.47869/tcsj.71.7.7
[19] C. Wilcox, W.L. Woon, Z. Aung, Applications of machine learning in environmental engineering, Citeseer, 2013. https://doi.org/10.1017/CBO9780511627217
[20] D.V. Dao, S.H. Trinh, H.-B. Ly, B.T. Pham, Prediction of compressive strength of geopolymer concrete using entirely steel slag aggregates: Novel hybrid artificial intelligence approaches, Applied Sciences, 9 (2019) 1113. https://doi.org/10.3390/app9061113
[21] S.J.S. Hakim, H.A. Razak, Structural damage detection of steel bridge girder using artificial neural networks and finite element models, Steel Compos. Struct, 14 (2013) 367-377. http://dx.doi.org/10.12989/scs.2013.14.4.367
[22] Z.-H. Duan, S.-C. Kou, C.-S. Poon, Prediction of compressive strength of recycled aggregate concrete using artificial neural networks, Construction and Building Materials, 40 (2013) 1200-1206. https://doi.org/10.1016/j.conbuildmat.2012.04.063
[23] K. Sahoo, P. Sarkar, P. Robin Davis, Artificial neural networks for prediction of compressive strength of recycled aggregate concrete, 1 (2016) 81-85. http://dx.doi.org/10.15242/IJRCMCE.IAE0316414
[24] N. Deshpande, S. Londhe, S. Kulkarni, Modeling compressive strength of recycled aggregate concrete by artificial neural network, model tree and non-linear regression, International Journal of Sustainable Built Environment, 3 (2014) 187-198. https://doi.org/10.1016/j.ijsbe.2014.12.002
[25] H.Q. Nguyen, H.B. Ly, V.Q. Tran, T.A. Nguyen, T.T. Le, B.T. Pham, Optimization of artificial intelligence system by evolutionary algorithm for prediction of axial capacity of rectangular concrete filled steel tubes under compression, Materials, 13 (2020). https://doi.org/10.3390/MA13051205
[26] S.A. Kalogirou, Applications of artificial neural-networks for energy systems, Applied Energy, 67 (2000) 17-35. https://doi.org/10.1016/S0306-2619(00)00005-2
[27] H.B. Ly, E. Monteiro, T.T. Le, V.M. Le, M. Dal, G. Regnier, B.T. Pham, Prediction and sensitivity analysis of bubble dissolution time in 3D selective laser sintering using ensemble decision trees, Materials, 12 (2019). https://doi.org/10.3390/ma12091544
[28] N. Long, F. Zhang, Novel Newton’s learning algorithm of neural networks, Journal of Systems Engineering and Electronics, 17 (2006) 450–454. https://doi.org/10.1016/S1004-4132(06)60076-5
[29] I. Livieris, P. Pintelas, A survey on algorithms for training artificial neural networks, 2008.
[30] P.S. Sandhu, S. Chhabra, A comparative analysis of conjugate gradient algorithms & PSO based neural network approaches for reusability evaluation of procedure based software systems, Chiang Mai Journal of Science, 38 (2011) 123–135
[31] A. Gholampour, A.H. Gandomi, T. Ozbakkaloglu, New formulations for mechanical properties of recycled aggregate concrete using gene expression programming, Construction and Building Materials, 130 (2017) 122–145. https://doi.org/10.1016/j.conbuildmat.2016.10.114
[32] I.H. Witten, E. Frank, Data mining: practical machine learning tools and techniques with Java implementations, Acm Sigmod Record. 31 (2002) 76–77
[33] H. Nguyen, H.-B. Ly, T. Van Quan, T.-A. Nguyen, T.-T. Le, B. Pham, Optimization of Artificial Intelligence System by Evolutionary Algorithm for Prediction of Axial Capacity of Rectangular Concrete Filled Steel Tubes under Compression, Materials. 13 (2020). https://doi.org/10.3390/ma13051205
[34] G. Cybenko, Approximation by superpositions of a sigmoidal function, Mathematics of Control, Signals and Systems, 2 (1989) 303–314
[35] D.G. Bounds, P.J. Lloyd, B.G. Mathew, G. Waddell, A multilayer perceptron network for the diagnosis of low back pain., in: ICNN, 1988 481-489
[36] J.-Z. Xiao, J.-B. Li, C. Zhang, On relationships between the mechanical properties of recycled aggregate concrete: an overview, Materials and Structures, 39 (2006) 655–664. https://doi.org/10.1617/s11527-006-9093-0
[37] P. Pereira, L. Evangelista, J. De Brito, The effect of superplasticisers on the workability and compressive strength of concrete made with fine recycled concrete aggregates, Construction and Building Materials, 28 (2012) 722–729. https://doi.org/10.1016/j.conbuildmat.2011.10.050
[38] C. Thomas, J. Setien, Ja. Polanco, P. Alaejos, M.S. De Juan, Durability of recycled aggregate concrete, Construction and Building Materials, 40 (2013) 1054–1065. https://doi.org/10.1016/j.conbuildmat.2012.11.106
[2] O. Cakır, Experimental analysis of properties of recycled coarse aggregate (RCA) concrete with mineral additives, Construction and Building Materials, 68 (2014) 17-25. https://doi.org/10.1016/j.conbuildmat.2014.06.032
[3] F. Pacheco-Torgal, Y. Ding, S. Miraldo, Z. Abdollahnejad, J.A. Labrincha, Are geopolymers more suitable than Portland cement to produce high volume recycled aggregates HPC?, Construction and Building Materials, 36 (2012) 1048-1052. https://doi.org/10.1016/j.conbuildmat.2012.07.004
[4] M. Ghanbari, A.M. Abbasi, M. Ravanshadnia, Production of natural and recycled aggregates: the environmental impacts of energy consumption and CO2 emissions, Journal of Material Cycles and Waste Management, 20 (2018) 810–822. https://doi.org/10.1007/s10163-017-0640-2
[5] M. Bravo, J. De Brito, J. Pontes, L. Evangelista, Mechanical performance of concrete made with aggregates from construction and demolition waste recycling plants, Journal of Cleaner Production, 99 (2015) 59-74. https://doi.org/10.1016/j.jclepro.2015.03.012
[6] B. Gonzalez-Fonteboa, F. Martinez-Abella, Concretes with aggregates from demolition waste and silica fume, Materials and mechanical properties, Building and Environment, 43 (2008) 429-437. https://doi.org/10.1016/j.buildenv.2007.01.008
[7] I.B. Topcu, M. Sarıdemir, Prediction of mechanical properties of recycled aggregate concretes containing silica fume using artificial neural networks and fuzzy logic, Computational Materials Science, 42 (2008) 74-82. https://doi.org/10.1016/j.commatsci.2007.06.011
[8] Z.H. Duan, C.S. Poon, Properties of recycled aggregate concrete made with recycled aggregates with different amounts of old adhered mortars, Materials & Design, 58 (2014) 19-29. https://doi.org/10.1016/j.matdes.2014.01.044
[9] D. Pedro, J. De Brito, L. Evangelista, Performance of concrete made with aggregates recycled from precasting industry waste: influence of the crushing process, Materials and Structures, 48 (2015) 3965-3978. https://doi.org/10.1617/s11527-014-0456-7
[10] R.V. Silva, J. De Brito, R.K. Dhir, The influence of the use of recycled aggregates on the compressive strength of concrete: a review, European Journal of Environmental and Civil Engineering, 19 (2015) 825-849. https://doi.org/10.1080/19648189.2014.974831
[11] K. Naouaoui, A. Bouyahyaoui, T. Cherradi, Experimental Characterization of Recycled Aggregate Concrete, in: MATEC Web of Conferences, EDP Sciences, 303 (2019) 05004. https://doi.org/10.1051/matecconf/201930305004
[12] K. Rahal, Mechanical properties of concrete with recycled coarse aggregate, Building and Environment, 42 (2007) 407-415. https://doi.org/10.1016/j.buildenv.2005.07.033
[13] T.-Y. Tu, Y.-Y. Chen, C.-L. Hwang, Properties of HPC with recycled aggregates, Cement and Concrete Research, 36 (2006) 943-950. https://doi.org/10.1016/j.cemconres.2005.11.022
[14] Q.H. Nguyen, H.-B. Ly, V.Q. Tran, T.-A. Nguyen, V.-H. Phan, T.-T. Le, B.T. Pham, A novel hybrid model based on a feedforward neural network and one step secant algorithm for prediction of load-bearing capacity of rectangular concrete-filled steel tube columns, Molecules. 25 (2020) 3486. https://doi.org/10.3390/molecules25153486
[15] Lý Hải Bằng, Nguyễn Thùy Anh, Nghiên cứu dự báo sức chịu tải tới hạn của cấu kiện cột ống thép nhồi bê tông có tiết diện hình chữ nhật bằng mạng nơ ron nhân tạo, Tạp chí Khoa học Giao thông vận tải, 71 (2020) 154-166. https://doi.org/ 10.25073/tcsj.71.2.10
[16] Nguyễn Thùy Anh, Lý Hải Bằng, Dự đoán sức kháng cắt của dầm bê tông cốt thanh FRP không có cốt thép đai sử dụng mạng thần kinh nhân tạo, Tạp chí Khoa học Giao thông vận tải, 71 (2020) 1047-1060. https://doi.org/10.47869/tcsj.71.9.4
[17] Nguyễn Thùy Anh, Lý Hải Bằng, Phương pháp dự đoán tải trọng ổn định đàn hồi của dầm thép H bản bụng khoét lỗ tròn dựa trên máy vec-tơ hỗ trợ, Tạp chí Khoa học Giao thông vận tải, 71 (2020) 500-513. https://doi.org/10.25073/tcsj.71.5.4
[18] Nguyễn Thuỳ Anh, Lý Hải Bằng, Development of ANN-based models to predict the bond strength of GFRP bars and concrete beams, Tạp chí Khoa học Giao thông vận tải, 71 (2020) 814-827. https://doi.org/10.47869/tcsj.71.7.7
[19] C. Wilcox, W.L. Woon, Z. Aung, Applications of machine learning in environmental engineering, Citeseer, 2013. https://doi.org/10.1017/CBO9780511627217
[20] D.V. Dao, S.H. Trinh, H.-B. Ly, B.T. Pham, Prediction of compressive strength of geopolymer concrete using entirely steel slag aggregates: Novel hybrid artificial intelligence approaches, Applied Sciences, 9 (2019) 1113. https://doi.org/10.3390/app9061113
[21] S.J.S. Hakim, H.A. Razak, Structural damage detection of steel bridge girder using artificial neural networks and finite element models, Steel Compos. Struct, 14 (2013) 367-377. http://dx.doi.org/10.12989/scs.2013.14.4.367
[22] Z.-H. Duan, S.-C. Kou, C.-S. Poon, Prediction of compressive strength of recycled aggregate concrete using artificial neural networks, Construction and Building Materials, 40 (2013) 1200-1206. https://doi.org/10.1016/j.conbuildmat.2012.04.063
[23] K. Sahoo, P. Sarkar, P. Robin Davis, Artificial neural networks for prediction of compressive strength of recycled aggregate concrete, 1 (2016) 81-85. http://dx.doi.org/10.15242/IJRCMCE.IAE0316414
[24] N. Deshpande, S. Londhe, S. Kulkarni, Modeling compressive strength of recycled aggregate concrete by artificial neural network, model tree and non-linear regression, International Journal of Sustainable Built Environment, 3 (2014) 187-198. https://doi.org/10.1016/j.ijsbe.2014.12.002
[25] H.Q. Nguyen, H.B. Ly, V.Q. Tran, T.A. Nguyen, T.T. Le, B.T. Pham, Optimization of artificial intelligence system by evolutionary algorithm for prediction of axial capacity of rectangular concrete filled steel tubes under compression, Materials, 13 (2020). https://doi.org/10.3390/MA13051205
[26] S.A. Kalogirou, Applications of artificial neural-networks for energy systems, Applied Energy, 67 (2000) 17-35. https://doi.org/10.1016/S0306-2619(00)00005-2
[27] H.B. Ly, E. Monteiro, T.T. Le, V.M. Le, M. Dal, G. Regnier, B.T. Pham, Prediction and sensitivity analysis of bubble dissolution time in 3D selective laser sintering using ensemble decision trees, Materials, 12 (2019). https://doi.org/10.3390/ma12091544
[28] N. Long, F. Zhang, Novel Newton’s learning algorithm of neural networks, Journal of Systems Engineering and Electronics, 17 (2006) 450–454. https://doi.org/10.1016/S1004-4132(06)60076-5
[29] I. Livieris, P. Pintelas, A survey on algorithms for training artificial neural networks, 2008.
[30] P.S. Sandhu, S. Chhabra, A comparative analysis of conjugate gradient algorithms & PSO based neural network approaches for reusability evaluation of procedure based software systems, Chiang Mai Journal of Science, 38 (2011) 123–135
[31] A. Gholampour, A.H. Gandomi, T. Ozbakkaloglu, New formulations for mechanical properties of recycled aggregate concrete using gene expression programming, Construction and Building Materials, 130 (2017) 122–145. https://doi.org/10.1016/j.conbuildmat.2016.10.114
[32] I.H. Witten, E. Frank, Data mining: practical machine learning tools and techniques with Java implementations, Acm Sigmod Record. 31 (2002) 76–77
[33] H. Nguyen, H.-B. Ly, T. Van Quan, T.-A. Nguyen, T.-T. Le, B. Pham, Optimization of Artificial Intelligence System by Evolutionary Algorithm for Prediction of Axial Capacity of Rectangular Concrete Filled Steel Tubes under Compression, Materials. 13 (2020). https://doi.org/10.3390/ma13051205
[34] G. Cybenko, Approximation by superpositions of a sigmoidal function, Mathematics of Control, Signals and Systems, 2 (1989) 303–314
[35] D.G. Bounds, P.J. Lloyd, B.G. Mathew, G. Waddell, A multilayer perceptron network for the diagnosis of low back pain., in: ICNN, 1988 481-489
[36] J.-Z. Xiao, J.-B. Li, C. Zhang, On relationships between the mechanical properties of recycled aggregate concrete: an overview, Materials and Structures, 39 (2006) 655–664. https://doi.org/10.1617/s11527-006-9093-0
[37] P. Pereira, L. Evangelista, J. De Brito, The effect of superplasticisers on the workability and compressive strength of concrete made with fine recycled concrete aggregates, Construction and Building Materials, 28 (2012) 722–729. https://doi.org/10.1016/j.conbuildmat.2011.10.050
[38] C. Thomas, J. Setien, Ja. Polanco, P. Alaejos, M.S. De Juan, Durability of recycled aggregate concrete, Construction and Building Materials, 40 (2013) 1054–1065. https://doi.org/10.1016/j.conbuildmat.2012.11.106
Tải xuống
Chưa có dữ liệu thống kê
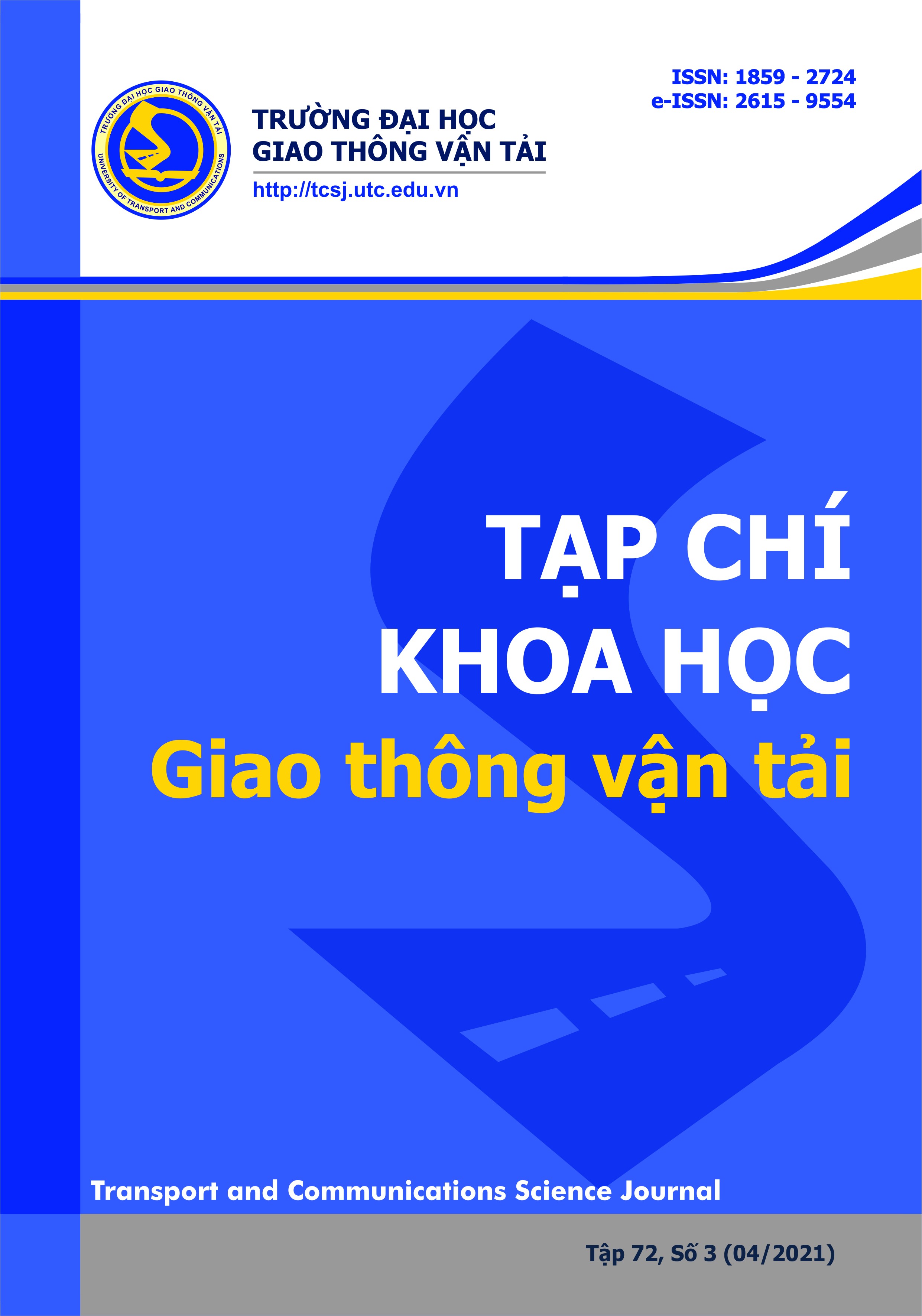
Nhận bài
28/01/2021
Nhận bài sửa
01/04/2021
Chấp nhận đăng
03/04/2021
Xuất bản
15/04/2021
Chuyên mục
Công trình khoa học
Kiểu trích dẫn
Lý Hải, B., Nguyễn Thùy, A., & Mai Thị Hải, V. (9600). Nghiên cứu ứng dụng mô hình trí tuệ nhân tạo dự báo cường độ nén của bê tông sử dụng cốt liệu tái chế. Tạp Chí Khoa Học Giao Thông Vận Tải, 72(3), 369-383. https://doi.org/10.47869/tcsj.72.3.11
Số lần xem tóm tắt
242
Số lần xem bài báo
512