Predicting the flexural capacity of corroded reinforced concrete beams using artificial intelligence models
Email:
ndinhhung@hcmiu.edu.vn
Từ khóa:
residual flexural capacity, corroded reinforced concrete beams, artificial intelligence, single model, combined model, repairing, strengthening
Tóm tắt
Predicting the residual flexural capacity of corroded reinforced concrete (RC) structures is to help civil engineers decide to repair or strengthen the structures. This study presents the application of six single algorithm-based models of artificial intelligence, such as artificial neural network (ANN), support vector machine (SVM), classification and regression trees (CART), linear regression (LR), general linear model (GENLIN), and automatic Chi-squared interaction detection (CHAID) to predict the residual flexural capacity of corroded RC structures. The predicting results are compared to the surveyed data including 120 corroded RC beams from the projects built before 1975 to rank the efficiency of single models. Some combined models are applied to investigate the improvement in predicting the flexural capacity of corroded RC structures compared to the single models. The result shows that LR and GENLIN models give almost the same results and the best efficiency. The combined models can not improve the efficiency compared to the two best single modelsTài liệu tham khảo
[1]. A. Imam, F. Anifowose, A. K. Azad, Residual Strength of Corroded Reinforced Concrete Beams Using an Adaptive Model Based on ANN, International Journal of Concrete Structures and Materials, 9 (2015) 159–172. https://doi.org/10.1007/s40069-015-0097-4
[2]. A. K. Azad, S. Ahmad, B. H. A. Al-Gohi, Flexural strength of corroded reinforced concrete beams, Magazine of Concrete Research, 62 (2010) 405-414. https://doi.org/10.1680/macr.2010.62.6.405
[3]. C.-H. Tsai, D.-S. Hsu, Diagnosis of Reinforced Concrete Structural Damage Base on Displacement Time History using the Back-Propagation Neural Network Technique, Journal of Computing in Civil Engineering, 16 (2002) 49–58. https://doi.org/10.1061/(ASCE)0887-3801(2002)16:1(49)
[4]. J.-S. Chou, N.-T. Ngo, W. K. Chong, The use of artificial intelligence combiners for modeling steel pitting risk and corrosion rate, Engineering Applications of Artificial Intelligence, 65 (2016) 471-483. https://doi.org/10.1016/j.engappai.2016.09.008
[5]. A. Imam, Z. A. Kazmi, Modified regression and ANN model for load carrying capacity of corroded reinforced concrete beam, AIMS Materials Science, 4 (2020) 1140-1164.
https://doi.org/10.3934/matersci.2017.5.1140
[6]. H. Alabduljabbar, J. H. Haido, R. Alyousef, S. T. Yousif, J. McConnell, K. Wakil, K. Jermsittiparsert, Prediction of the flexural behavior of corroded concrete beams using combined method, Structures, 25 (2020) 1000-1008. https://doi.org/10.1016/j.istruc.2020.03.057
[7]. M. Ahmadi, A. Kheyroddin, M. Kioumarsi, Prediction models for bond strength of steel reinforcement with consideration of corrosion, Materials Today: Proceedings, 2021. https://doi.org/10.1016/j.matpr.2021.03.263
[8]. M. E. A. Ben Seghier, B. Keshtegar, H. Mahmoud, Time-Dependent Reliability Analysis of Reinforced Concrete Beams Subjected to Uniform and Pitting Corrosion and Brittle Fracture, Materials, 14 (2021) 1820. https://doi.org/10.3390/ma14081820
[9]. C. A. Jeyasehar, K. Sumangala, Nondestructive Evaluation of Prestressed Concrete Beams using an Artificial Neural Network (ANN) Approach, Structural Health Monitoring: An International Journal, 5(2006) 313-323. https://doi.org/10.1177/1475921706067759
[10]. J. A. Abdalla, A. Elsanosi, A. Abdelwahab, Modeling and simulation of shear resistance of R/C beams using artificial neural network, Journal of the Franklin Institute, 344 (2007) 741-756. https://doi.org/10.1016/j.jfranklin.2005.12.005
[11] A. Imam, A. K. Azad, Prediction of residual shear strength of corroded reinforced concrete beams, International Journal of Advanced Structural Engineering, 8 (2016) 307-318. https://doi.org/10.1007/s40091-016-0133-x
[12] E. P. Kumar, E. P. Sharma, Artificial Neural Networks-A Study, International Journal of Emerging Engineering Research and Technology, 2 (2014) 143-148.
[13] A. M. Deris, A. M. Zain, R. Sallehuddin, Overview of Support Vector Machine in Modeling Machining Performances, Procedia Engineering, 24 (2011) 308-312. https://doi.org/10.1016/j.proeng.2011.11.2647
[14] T. D. Hoc, Predicting the grouting ability of self-suction fine cement mortar based on artificial intelligence techniques, Vietnam Journal of Construction, 3 (2020) 118-120.
[15] L. Rutkowski, M. Jaworski, L. Pietruczuk, P. Duda, The CART Decision Tree for Mining Data Streams, Information Sciences, 266 (2014) 1-15.
[16] J.-S. Chou, A.-D. Pham, Enhanced artificial intelligence for ensemble approach to predicting high-performance concrete compressive strength, Construction and Building Materials, 49 (2013) 554-563. https://doi.org/10.1016/j.conbuildmat.2013.08.078
[17] J. A. Nelder, R. W. M. Wedderburn, Generalized Linear Models, Journal of the Royal Statistical Society: Series A (General), 135 (1972) 370-384. https://www.jstor.org/stable/2344614
[18] G. V. Kass, An Exploratory Technique for Investigating Large Quantities of Categorical Data, Journal of the Royal Statistical Society: Series C (Applied Statistics), 29(1980) 119–127. https://doi.org/10.2307/2986296
[19] D. Biggs, B. De Ville, E. Suen, A method of choosing multiway partitions for classification and decision trees, Journal of Applied Statistics, 18 (1991) 49-62. http://dx.doi.org/10.1080/02664769100000005
[20] P. J. L. Adeodato, A. L. Arnaud, G. C. Vasconcelos, R. C. L. V. Cunha, D. S. M. P. Monteiro, MLP ensembles improve long-term prediction accuracy over single networks, International Journal of Forecasting, 27 (2011) 661-671. https://doi.org/10.1016/j.ijforecast.2009.05.029
[21] M. van Wezel, R. Potharst, Improved customer choice predictions using ensemble methods, European Journal of Operational Research, 181 (2007) 436-452.
[22] Center for Housing Management and Construction Inspection of Ho Chi Minh City Department of Construction: Report on the inspection of reinforced concrete corroded beams of apartments and collective residences, 2016.
[23] TCVN 9334:2012, Heavy concrete – Method for determining compressive strength by a bouncing gun Ministry of Science and Technology, Vietnam, 2012.
[24] TCVN 9357:2012, Heavy concrete – Non-destructive testing method – Evaluation of concrete quality by ultrasonic pulse velocity, Ministry of Science and Technology, Vietnam, 2012.
[25] TCVN 3105:1993, Mixture of heavy concrete and heavy concrete - Sampling, fabrication and curing of specimens, Ministry of Science and Technology, Vietnam, 1993.
[26] TCVN 3118:1993 Heavy concrete – Method for determining compressive strength, Ministry of Science and Technology, Vietnam, 1993.
[27] TCXDVN 239:2006, Heavy concrete - Guidelines for assessing concrete strength on building structures, Ministry of Science and Technology, Vietnam, 2006.
[28] TCVN 8634:2010 Callipers up to 0.02mm - Standard Measurement and Quality, Ministry of Science and Technology, Vietnam, 2010.
[29] TCVN 4101:1985 Measuring scale - technical requirements - Standards, metrology and quality, Ministry of Science and Technology, Vietnam, 1985.
[30] TCVN 2737:1995 loads and impacts - design standards, Ministry of Science and Technology, Vietnam, 1995.
[31] TCVN 5574:2018 design of concrete and reinforced concrete structures, Ministry of Science and Technology, Vietnam, 2018.
[32] https://drive.google.com/file/d/1SO_z0Ceu-WzX7qPMQueu9G2GVTFNqlbD/view?usp=sharing.
[2]. A. K. Azad, S. Ahmad, B. H. A. Al-Gohi, Flexural strength of corroded reinforced concrete beams, Magazine of Concrete Research, 62 (2010) 405-414. https://doi.org/10.1680/macr.2010.62.6.405
[3]. C.-H. Tsai, D.-S. Hsu, Diagnosis of Reinforced Concrete Structural Damage Base on Displacement Time History using the Back-Propagation Neural Network Technique, Journal of Computing in Civil Engineering, 16 (2002) 49–58. https://doi.org/10.1061/(ASCE)0887-3801(2002)16:1(49)
[4]. J.-S. Chou, N.-T. Ngo, W. K. Chong, The use of artificial intelligence combiners for modeling steel pitting risk and corrosion rate, Engineering Applications of Artificial Intelligence, 65 (2016) 471-483. https://doi.org/10.1016/j.engappai.2016.09.008
[5]. A. Imam, Z. A. Kazmi, Modified regression and ANN model for load carrying capacity of corroded reinforced concrete beam, AIMS Materials Science, 4 (2020) 1140-1164.
https://doi.org/10.3934/matersci.2017.5.1140
[6]. H. Alabduljabbar, J. H. Haido, R. Alyousef, S. T. Yousif, J. McConnell, K. Wakil, K. Jermsittiparsert, Prediction of the flexural behavior of corroded concrete beams using combined method, Structures, 25 (2020) 1000-1008. https://doi.org/10.1016/j.istruc.2020.03.057
[7]. M. Ahmadi, A. Kheyroddin, M. Kioumarsi, Prediction models for bond strength of steel reinforcement with consideration of corrosion, Materials Today: Proceedings, 2021. https://doi.org/10.1016/j.matpr.2021.03.263
[8]. M. E. A. Ben Seghier, B. Keshtegar, H. Mahmoud, Time-Dependent Reliability Analysis of Reinforced Concrete Beams Subjected to Uniform and Pitting Corrosion and Brittle Fracture, Materials, 14 (2021) 1820. https://doi.org/10.3390/ma14081820
[9]. C. A. Jeyasehar, K. Sumangala, Nondestructive Evaluation of Prestressed Concrete Beams using an Artificial Neural Network (ANN) Approach, Structural Health Monitoring: An International Journal, 5(2006) 313-323. https://doi.org/10.1177/1475921706067759
[10]. J. A. Abdalla, A. Elsanosi, A. Abdelwahab, Modeling and simulation of shear resistance of R/C beams using artificial neural network, Journal of the Franklin Institute, 344 (2007) 741-756. https://doi.org/10.1016/j.jfranklin.2005.12.005
[11] A. Imam, A. K. Azad, Prediction of residual shear strength of corroded reinforced concrete beams, International Journal of Advanced Structural Engineering, 8 (2016) 307-318. https://doi.org/10.1007/s40091-016-0133-x
[12] E. P. Kumar, E. P. Sharma, Artificial Neural Networks-A Study, International Journal of Emerging Engineering Research and Technology, 2 (2014) 143-148.
[13] A. M. Deris, A. M. Zain, R. Sallehuddin, Overview of Support Vector Machine in Modeling Machining Performances, Procedia Engineering, 24 (2011) 308-312. https://doi.org/10.1016/j.proeng.2011.11.2647
[14] T. D. Hoc, Predicting the grouting ability of self-suction fine cement mortar based on artificial intelligence techniques, Vietnam Journal of Construction, 3 (2020) 118-120.
[15] L. Rutkowski, M. Jaworski, L. Pietruczuk, P. Duda, The CART Decision Tree for Mining Data Streams, Information Sciences, 266 (2014) 1-15.
[16] J.-S. Chou, A.-D. Pham, Enhanced artificial intelligence for ensemble approach to predicting high-performance concrete compressive strength, Construction and Building Materials, 49 (2013) 554-563. https://doi.org/10.1016/j.conbuildmat.2013.08.078
[17] J. A. Nelder, R. W. M. Wedderburn, Generalized Linear Models, Journal of the Royal Statistical Society: Series A (General), 135 (1972) 370-384. https://www.jstor.org/stable/2344614
[18] G. V. Kass, An Exploratory Technique for Investigating Large Quantities of Categorical Data, Journal of the Royal Statistical Society: Series C (Applied Statistics), 29(1980) 119–127. https://doi.org/10.2307/2986296
[19] D. Biggs, B. De Ville, E. Suen, A method of choosing multiway partitions for classification and decision trees, Journal of Applied Statistics, 18 (1991) 49-62. http://dx.doi.org/10.1080/02664769100000005
[20] P. J. L. Adeodato, A. L. Arnaud, G. C. Vasconcelos, R. C. L. V. Cunha, D. S. M. P. Monteiro, MLP ensembles improve long-term prediction accuracy over single networks, International Journal of Forecasting, 27 (2011) 661-671. https://doi.org/10.1016/j.ijforecast.2009.05.029
[21] M. van Wezel, R. Potharst, Improved customer choice predictions using ensemble methods, European Journal of Operational Research, 181 (2007) 436-452.
[22] Center for Housing Management and Construction Inspection of Ho Chi Minh City Department of Construction: Report on the inspection of reinforced concrete corroded beams of apartments and collective residences, 2016.
[23] TCVN 9334:2012, Heavy concrete – Method for determining compressive strength by a bouncing gun Ministry of Science and Technology, Vietnam, 2012.
[24] TCVN 9357:2012, Heavy concrete – Non-destructive testing method – Evaluation of concrete quality by ultrasonic pulse velocity, Ministry of Science and Technology, Vietnam, 2012.
[25] TCVN 3105:1993, Mixture of heavy concrete and heavy concrete - Sampling, fabrication and curing of specimens, Ministry of Science and Technology, Vietnam, 1993.
[26] TCVN 3118:1993 Heavy concrete – Method for determining compressive strength, Ministry of Science and Technology, Vietnam, 1993.
[27] TCXDVN 239:2006, Heavy concrete - Guidelines for assessing concrete strength on building structures, Ministry of Science and Technology, Vietnam, 2006.
[28] TCVN 8634:2010 Callipers up to 0.02mm - Standard Measurement and Quality, Ministry of Science and Technology, Vietnam, 2010.
[29] TCVN 4101:1985 Measuring scale - technical requirements - Standards, metrology and quality, Ministry of Science and Technology, Vietnam, 1985.
[30] TCVN 2737:1995 loads and impacts - design standards, Ministry of Science and Technology, Vietnam, 1995.
[31] TCVN 5574:2018 design of concrete and reinforced concrete structures, Ministry of Science and Technology, Vietnam, 2018.
[32] https://drive.google.com/file/d/1SO_z0Ceu-WzX7qPMQueu9G2GVTFNqlbD/view?usp=sharing.
Tải xuống
Chưa có dữ liệu thống kê
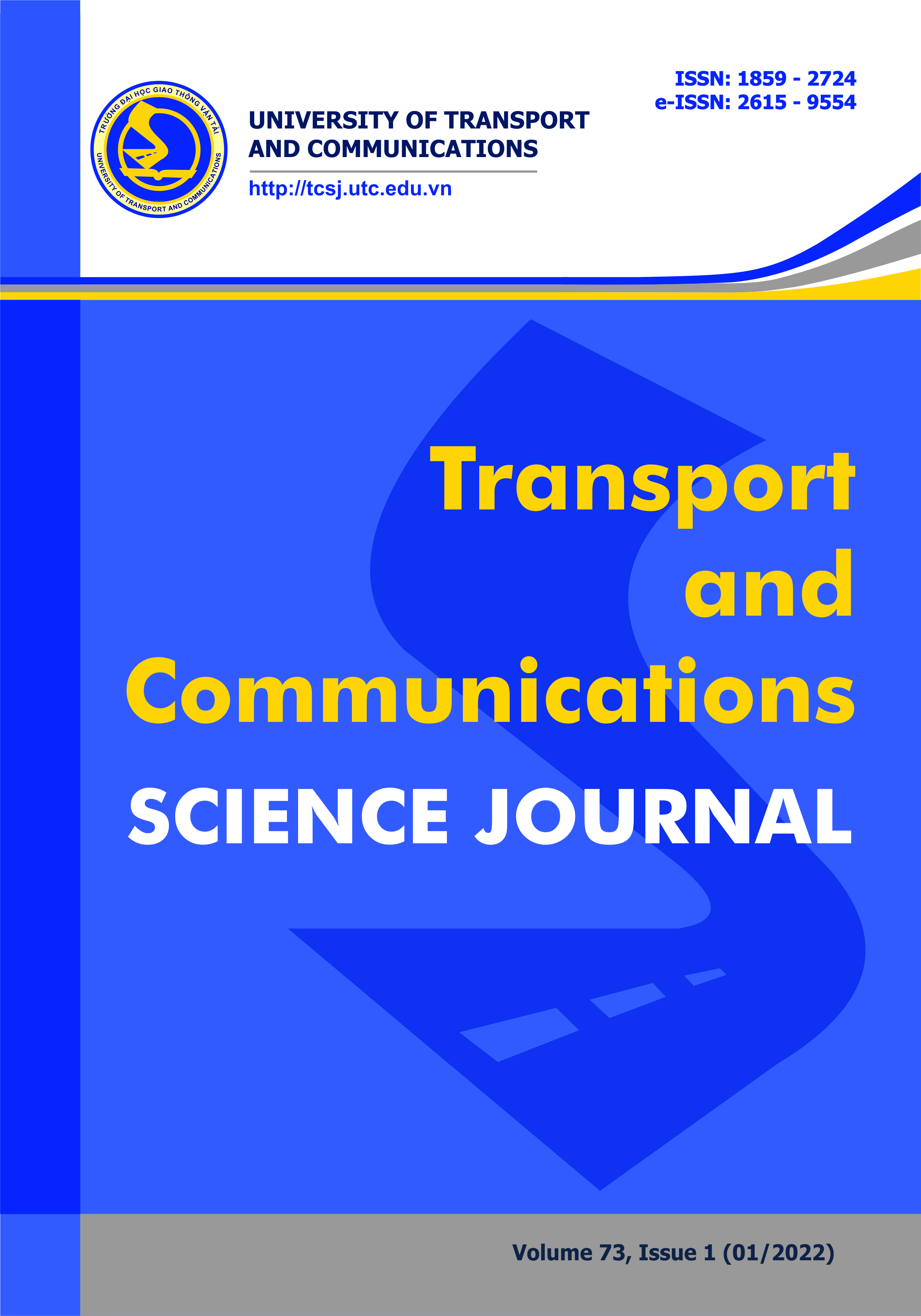
Nhận bài
30/08/2021
Nhận bài sửa
04/11/2021
Chấp nhận đăng
12/11/2021
Xuất bản
15/01/2022
Chuyên mục
Công trình khoa học
Kiểu trích dẫn
Nguyen Thanh, H., Le Minh, C., Doan Dinh Thien, V., & Nguyen Dinh, H. (1642179600). Predicting the flexural capacity of corroded reinforced concrete beams using artificial intelligence models. Tạp Chí Khoa Học Giao Thông Vận Tải, 73(1), 40-51. https://doi.org/10.47869/tcsj.73.1.4
Số lần xem tóm tắt
380
Số lần xem bài báo
547