Nghiên cứu ứng dụng mô hình mạng nơ ron nhân tạo dự báo mô đun đàn hồi động của vật liệu đất đắp nền đường
Email:
banglh@utt.edu.vn
Từ khóa:
Mô đun đàn hồi, vật liệu đất đắp nền đường, mô hình mạng nơ ron nhân tạo (ANN), thuật toán One-step-secant (OSS)
Tóm tắt
Kết cấu nền – mặt đường ô tô bao gồm các lớp mặt đường và nền đất, trong đó, tác dụng chính của nền là phân bố, giảm ứng suất dọc từ trên mặt đường xuống khi chịu tác dụng của tải trọng. Để đảm bảo cường độ và ổn định của kết cấu nền – mặt đường, nền đất phải có đủ cường độ. Đặc trưng cho cường độ của đất nền là mô đun đàn hồi, là một thông số quan trọng sử dụng trong tính toán, kiểm toán kết cấu nền - mặt đường. Tuy nhiên, việc xác định mô đun đàn hồi của vật liệu đất đắp nền đường bằng các phương pháp thí nghiệm trong phòng hay thực nghiệm ngoài hiện trường như hiện nay còn công phu, thiết bị thí nghiệm cồng kềnh, tốn kém về thời gian, chi phí. Trong nghiên cứu này, mô hình mạng nơ ron nhân tạo với thuật toán One-step-secant được đề xuất để dự đoán mô đun đàn hồi của vật liệu đất nền. Cơ sở dữ liệu trong nghiên cứu gồm 418 kết quả thí nghiệm được sử dụng để phát triển mô hình mạng nơ ron nhân tạo (ANN). Kết quả cho thấy mô hình ANN là một công cụ hiệu quả trong việc dự đoán mô đun đàn hồi đất nền (RMSE=2.9401, MAE=2.3075, R2=0.9858), giúp tiết kiệm thời gian, giảm chi phí thí nghiệm. Ngoài ra, mô hình ANN còn đánh giá được mức độ ảnh hưởng của từng tham số đến mô đun đàn hồi của vật liệu đất đắp nền đường, trong đó ứng suất giới hạn là thông số quan trọng nhất.Tài liệu tham khảo
[1] W. Hanittinan, Resilient Modulus Prediction Using Neural Network Algorithm, Ohio State University, (2007). http://rave.ohiolink.edu/etdc/view?acc_num=osu1190140082
[2] Y. Huang, Pavement Analysis and Design, 2nd edition, Pearson, Upper Saddle River, NJ, (2003). http://testbank360.eu/solution-manual-pavement-analysis-and-design-2nd-edition-huang
[3] W. Lee, N.C. Bohra, A. G. Altschaeffl, T. D. White, Resilient Modulus of Cohesive Soils, Journal of Geotechnical and Geoenvironmental Engineering, 123 (1997) 131-136. https://doi.org/10.1061/(ASCE)1090-0241(1997)123:2(131)
[4] C. Olidid, D. Hein, Guide for the Mechanistic-Empirical Design of New and Rehabilitated Pavement Structures, (2004). https://trid.trb.org/view/768322
[5] E. C. Drumm, Y. Boateng‐Poku, T. Johnson Pierce, Estimation of Subgrade Resilient Modulus from Standard Tests, Journal of Geotechnical Engineering, 116 (1990) 774-789. https://doi.org/10.1061/(ASCE)0733-9410(1990)116:5(774)
[6] D. Andrei, M. W. Witczak, C. W. Schwartz, J. Uzan, Harmonized Resilient Modulus Test Method for Unbound Pavement Materials, Transportation Research Record, 1874 (2004) 29-37. https://doi.org/10.3141/1874-04
[7] D. Kim, J. R. Kim, Resilient behavior of compacted subgrade soils under the repeated triaxial test, Construction and Building Materials, 21 (2007) 1470-1479. https://doi.org/10.1016/j.conbuildmat.2006.07.006
[8] M. Mazari, E. Navarro, I. Abdallah, S. Nazarian, Comparison of numerical and experimental responses of pavement systems using various resilient modulus models, Soils and Foundations, 54 (2014) 36-44. https://doi.org/10.1016/j.sandf.2013.12.004
[9] Nguyễn Quang Tiến, Thực nghiệm đánh giá mô đun đàn hồi động của vật liệu đắt đắp nền đường trên cao tốc Nội Bài - Lào Cai, Tạp Chí Giao thông vận tải, (2018).
[10] American Association of State Highway and Transportation Officials, AASHTO Guide for Design of Pavement Structures, (1993).
[11] Tiêu chuẩn quốc gia, TCVN 8861:2011: Áo đường mềm - Xác định mô đun đàn hồi của nền đất và các lớp kết cấu áo đường bằng phương pháp sử dụng tấm ép cứng, (2011).
[12] Bộ Giao thông vận tải, 22TCN 335-06: Xác định mô đun đàn hồi của nền đường và mô đun đàn hồi hữu hiệu của áo đường mềm bằng thiết bị đo độ võng FWD, (2006).
[13] H. I. Park, G. C. Kweon, S. R. Lee, Prediction of Resilient Modulus of Granular Subgrade Soils and Subbase Materials using Artificial Neural Network, Road Materials and Pavement Design, 10 (2009) 647-665. https://doi.org/10.1080/14680629.2009.9690218
[14] N. N. Khoury, Durability of cementitiously stabilized aggregate bases for pavement application, (2005). https://hdl.handle.net/11244/950
[15] N. Khoury, M. M. Zaman, Durability of stabilized base courses subjected to wet–dry cycles, International Journal of Pavement Engineering, 8 (2007) 265-276. https://doi.org/10.1080/10298430701342874
[16] M. Y. Mansour, M. Dicleli, J. Y. Lee, J. Zhang, Predicting the shear strength of reinforced concrete beams using artificial neural networks, Engineering Structures, 26 (2004) 781-799. https://doi.org/10.1016/j.engstruct.2004.01.011
[17] C. Avila, Y. Shiraishi, Y. Tsuji, Crack width prediction of reinforced concrete structures by artificial neural networks, in: 7th Seminar on Neural Network Applications in Electrical Engineering, (2004) 39-44. https://doi.org/10.1109/NEUREL.2004.1416529
[18] T.-A. Nguyen, H.-B. Ly, H.-V.T. Mai, V.Q. Tran, Prediction of Later-Age Concrete Compressive Strength Using Feedforward Neural Network, Advances in Materials Science and Engineering, (2020) 1-8. https://doi.org/10.1155/2020/9682740
[19] F. Khademi, S. M. Jamal, N. Deshpande, S. Londhe, Predicting strength of recycled aggregate concrete using Artificial Neural Network, Adaptive Neuro-Fuzzy Inference System and Multiple Linear Regression, International Journal of Sustainable Built Environment, 5 (2016) 355-369. https://doi.org/10.1016/j.ijsbe.2016.09.003
[20] İ. B. Topçu, M. Sarıdemir, Prediction of compressive strength of concrete containing fly ash using artificial neural networks and fuzzy logic, Computational Materials Science, 41 (2008) 305-311. https://doi.org/10.1016/j.commatsci.2007.04.009
[21] B. K. R. Prasad, H. Eskandari, B. V. V. Reddy, Prediction of compressive strength of SCC and HPC with high volume fly ash using ANN, Construction and Building Materials, 23 (2009) 117-128. https://doi.org/10.1016/j.conbuildmat.2008.01.014
[22] F. Özcan, C. D. Atiş, O. Karahan, E. Uncuoğlu, H. Tanyildizi, Comparison of artificial neural network and fuzzy logic models for prediction of long-term compressive strength of silica fume concrete, Advances in Engineering Software, 40 (2009) 856-863. https://doi.org/10.1016/j.advengsoft.2009.01.005
[23] D. V. Dao, H.-B. Ly, S. H. Trinh, T.-T. Le, B. T. Pham, Artificial Intelligence Approaches for Prediction of Compressive Strength of Geopolymer Concrete, Materials (Basel), 12 (2019). https://doi.org/10.3390/ma12060983
[24] Vu Van Tuan, Artificial neural network (ANN) model in predicting multi-layered ground settlements of metro tunnel, Tạp chí KHCN xây dựng, 4 (2019) 58-63
[25] M. Shahin, M. Jaksa, H. Maier, Artificial Neural Network Applications in Geotechnical Engineering, Australian Geomechanics, 36 (2001) 49-62. https://www.researchgate.net/publication/245498369
[26] M. A. Shahin, M. B. Jaksa, H. R. Maier, Recent Advances and Future Challenges for Artificial Neural Systems in Geotechnical Engineering Applications, Advances in Artificial Neural Systems, (2009) 1-9. https://doi.org/10.1155/2009/308239
[27] Vũ Văn Tuấn, Lựa chọn cấu trúc mạng nơ ron nhân tạo (ANN) dự báo chỉ số nén của đất, Tạp chí KHCN xây dựng, 3 (2020) 67-75
[28] R. W. Meier, G. J. Rix, Backcalculation of flexible pavement moduli using artificial neural networks, Transportation Research Record, (1994) 75-82. http://onlinepubs.trb.org/Onlinepubs/trr/1994/1448/1448-010.pdf
[29] S. Bredenhann, M. Ven, Application of artificial neural networks in te back-calculation of flexible pavement layer moduli from deflection measurements, (2004). https://www.researchgate.net/publication/251855389
[30] Y. M. A. Hashash, J. Ghaboussi, Q. Fu, C. Marulanda, Constitutive Soil Behavior Representation via Artificial Neural Networks: A Shift from Soil Models to Soil Behavior Data, (2012) 1-6. https://doi.org/10.1061/40803(187)126
[31] M. B. Bayrak, A. Guclu, H. Ceylan, Rapid Pavement Backcalculation Technique for Evaluating Flexible Pavement Systems, (2005). https://trid.trb.org/view/760277
[32] J. V Tu, Advantages and disadvantages of using artificial neural networks versus logistic regression for predicting medical outcomes, J. Clin. Epidemiol., 49 (1996) 1225-1231. https://doi.org/10.1016/S0895-4356(96)00002-9. (n.d.)
[33] Q. H. Nguyen, H.-B. Ly, V.Q. Tran, T.-A. Nguyen, V.-H. Phan, T.-T. Le, B. T. Pham, A Novel Hybrid Model Based on a Feedforward Neural Network and One Step Secant Algorithm for Prediction of Load-Bearing Capacity of Rectangular Concrete-Filled Steel Tube Columns, Molecules, 25 (2020) 3486. https://doi.org/10.3390/molecules25153486
[34] H.-B. Ly, L. M. Le, H. T. Duong, T. C. Nguyen, T. A. Pham, T.-T. Le, V. M. Le, L. Nguyen-Ngoc, B. T. Pham, Hybrid artificial intelligence approaches for predicting critical buckling load of structural members under compression considering the influence of initial geometric imperfections, Applied Sciences, 9 (2019) 2258. https://doi.org/10.3390/app9112258
[35] D.V. Dao, S. H. Trinh, H.-B. Ly, B. T. Pham, Prediction of compressive strength of geopolymer concrete using entirely steel slag aggregates: Novel hybrid artificial intelligence approaches, Applied Sciences, 9 (2019) 1113. https://doi.org/10.3390/app9061113
[36] D. V. Dao, H. Adeli, H.-B. Ly, L. M. Le, V. M. Le, T.-T. Le, B. T. Pham, A sensitivity and robustness analysis of GPR and ANN for high-performance concrete compressive strength prediction using a Monte Carlo simulation, Sustainability, 12 (2020) 830. https://doi.org/10.3390/su12030830
[37] K. G. Sheela, S. N. Deepa, Review on methods to fix number of hidden neurons in neural networks, Mathematical Problems in Engineering, 2013 (2013). https://doi.org/10.1155/2013/425740
[38] P. B. Cachim, Using artificial neural networks for calculation of temperatures in timber under fire loading, Construction and Building Materials, 25 (2011) 4175-4180. https://doi.org/10.1016/j.conbuildmat.2011.04.054
[39] G. Cybenko, Approximation by superpositions of a sigmoidal function, Mathematics of Control, Signals and Systems, 2 (1989) 303–314
[40] D. G. Bounds, P. J. Lloyd, B. G. Mathew, G. Waddell, A multilayer perceptron network for the diagnosis of low back pain., in: ICNN, (1988) 481-489. https://doi.org/10.1109/ICNN.1988.23963
[41] S. Nagendra, Practical Aspects of Using Neural Networks: Necessary Preliminary Specifications, Technical Paper, GE Research and Development Center, (1998).
[42] K. P. George, Prediction of resilient modulus from soil index properties, University of Mississippi, (2004). https://rosap.ntl.bts.gov/view/dot/16187
[2] Y. Huang, Pavement Analysis and Design, 2nd edition, Pearson, Upper Saddle River, NJ, (2003). http://testbank360.eu/solution-manual-pavement-analysis-and-design-2nd-edition-huang
[3] W. Lee, N.C. Bohra, A. G. Altschaeffl, T. D. White, Resilient Modulus of Cohesive Soils, Journal of Geotechnical and Geoenvironmental Engineering, 123 (1997) 131-136. https://doi.org/10.1061/(ASCE)1090-0241(1997)123:2(131)
[4] C. Olidid, D. Hein, Guide for the Mechanistic-Empirical Design of New and Rehabilitated Pavement Structures, (2004). https://trid.trb.org/view/768322
[5] E. C. Drumm, Y. Boateng‐Poku, T. Johnson Pierce, Estimation of Subgrade Resilient Modulus from Standard Tests, Journal of Geotechnical Engineering, 116 (1990) 774-789. https://doi.org/10.1061/(ASCE)0733-9410(1990)116:5(774)
[6] D. Andrei, M. W. Witczak, C. W. Schwartz, J. Uzan, Harmonized Resilient Modulus Test Method for Unbound Pavement Materials, Transportation Research Record, 1874 (2004) 29-37. https://doi.org/10.3141/1874-04
[7] D. Kim, J. R. Kim, Resilient behavior of compacted subgrade soils under the repeated triaxial test, Construction and Building Materials, 21 (2007) 1470-1479. https://doi.org/10.1016/j.conbuildmat.2006.07.006
[8] M. Mazari, E. Navarro, I. Abdallah, S. Nazarian, Comparison of numerical and experimental responses of pavement systems using various resilient modulus models, Soils and Foundations, 54 (2014) 36-44. https://doi.org/10.1016/j.sandf.2013.12.004
[9] Nguyễn Quang Tiến, Thực nghiệm đánh giá mô đun đàn hồi động của vật liệu đắt đắp nền đường trên cao tốc Nội Bài - Lào Cai, Tạp Chí Giao thông vận tải, (2018).
[10] American Association of State Highway and Transportation Officials, AASHTO Guide for Design of Pavement Structures, (1993).
[11] Tiêu chuẩn quốc gia, TCVN 8861:2011: Áo đường mềm - Xác định mô đun đàn hồi của nền đất và các lớp kết cấu áo đường bằng phương pháp sử dụng tấm ép cứng, (2011).
[12] Bộ Giao thông vận tải, 22TCN 335-06: Xác định mô đun đàn hồi của nền đường và mô đun đàn hồi hữu hiệu của áo đường mềm bằng thiết bị đo độ võng FWD, (2006).
[13] H. I. Park, G. C. Kweon, S. R. Lee, Prediction of Resilient Modulus of Granular Subgrade Soils and Subbase Materials using Artificial Neural Network, Road Materials and Pavement Design, 10 (2009) 647-665. https://doi.org/10.1080/14680629.2009.9690218
[14] N. N. Khoury, Durability of cementitiously stabilized aggregate bases for pavement application, (2005). https://hdl.handle.net/11244/950
[15] N. Khoury, M. M. Zaman, Durability of stabilized base courses subjected to wet–dry cycles, International Journal of Pavement Engineering, 8 (2007) 265-276. https://doi.org/10.1080/10298430701342874
[16] M. Y. Mansour, M. Dicleli, J. Y. Lee, J. Zhang, Predicting the shear strength of reinforced concrete beams using artificial neural networks, Engineering Structures, 26 (2004) 781-799. https://doi.org/10.1016/j.engstruct.2004.01.011
[17] C. Avila, Y. Shiraishi, Y. Tsuji, Crack width prediction of reinforced concrete structures by artificial neural networks, in: 7th Seminar on Neural Network Applications in Electrical Engineering, (2004) 39-44. https://doi.org/10.1109/NEUREL.2004.1416529
[18] T.-A. Nguyen, H.-B. Ly, H.-V.T. Mai, V.Q. Tran, Prediction of Later-Age Concrete Compressive Strength Using Feedforward Neural Network, Advances in Materials Science and Engineering, (2020) 1-8. https://doi.org/10.1155/2020/9682740
[19] F. Khademi, S. M. Jamal, N. Deshpande, S. Londhe, Predicting strength of recycled aggregate concrete using Artificial Neural Network, Adaptive Neuro-Fuzzy Inference System and Multiple Linear Regression, International Journal of Sustainable Built Environment, 5 (2016) 355-369. https://doi.org/10.1016/j.ijsbe.2016.09.003
[20] İ. B. Topçu, M. Sarıdemir, Prediction of compressive strength of concrete containing fly ash using artificial neural networks and fuzzy logic, Computational Materials Science, 41 (2008) 305-311. https://doi.org/10.1016/j.commatsci.2007.04.009
[21] B. K. R. Prasad, H. Eskandari, B. V. V. Reddy, Prediction of compressive strength of SCC and HPC with high volume fly ash using ANN, Construction and Building Materials, 23 (2009) 117-128. https://doi.org/10.1016/j.conbuildmat.2008.01.014
[22] F. Özcan, C. D. Atiş, O. Karahan, E. Uncuoğlu, H. Tanyildizi, Comparison of artificial neural network and fuzzy logic models for prediction of long-term compressive strength of silica fume concrete, Advances in Engineering Software, 40 (2009) 856-863. https://doi.org/10.1016/j.advengsoft.2009.01.005
[23] D. V. Dao, H.-B. Ly, S. H. Trinh, T.-T. Le, B. T. Pham, Artificial Intelligence Approaches for Prediction of Compressive Strength of Geopolymer Concrete, Materials (Basel), 12 (2019). https://doi.org/10.3390/ma12060983
[24] Vu Van Tuan, Artificial neural network (ANN) model in predicting multi-layered ground settlements of metro tunnel, Tạp chí KHCN xây dựng, 4 (2019) 58-63
[25] M. Shahin, M. Jaksa, H. Maier, Artificial Neural Network Applications in Geotechnical Engineering, Australian Geomechanics, 36 (2001) 49-62. https://www.researchgate.net/publication/245498369
[26] M. A. Shahin, M. B. Jaksa, H. R. Maier, Recent Advances and Future Challenges for Artificial Neural Systems in Geotechnical Engineering Applications, Advances in Artificial Neural Systems, (2009) 1-9. https://doi.org/10.1155/2009/308239
[27] Vũ Văn Tuấn, Lựa chọn cấu trúc mạng nơ ron nhân tạo (ANN) dự báo chỉ số nén của đất, Tạp chí KHCN xây dựng, 3 (2020) 67-75
[28] R. W. Meier, G. J. Rix, Backcalculation of flexible pavement moduli using artificial neural networks, Transportation Research Record, (1994) 75-82. http://onlinepubs.trb.org/Onlinepubs/trr/1994/1448/1448-010.pdf
[29] S. Bredenhann, M. Ven, Application of artificial neural networks in te back-calculation of flexible pavement layer moduli from deflection measurements, (2004). https://www.researchgate.net/publication/251855389
[30] Y. M. A. Hashash, J. Ghaboussi, Q. Fu, C. Marulanda, Constitutive Soil Behavior Representation via Artificial Neural Networks: A Shift from Soil Models to Soil Behavior Data, (2012) 1-6. https://doi.org/10.1061/40803(187)126
[31] M. B. Bayrak, A. Guclu, H. Ceylan, Rapid Pavement Backcalculation Technique for Evaluating Flexible Pavement Systems, (2005). https://trid.trb.org/view/760277
[32] J. V Tu, Advantages and disadvantages of using artificial neural networks versus logistic regression for predicting medical outcomes, J. Clin. Epidemiol., 49 (1996) 1225-1231. https://doi.org/10.1016/S0895-4356(96)00002-9. (n.d.)
[33] Q. H. Nguyen, H.-B. Ly, V.Q. Tran, T.-A. Nguyen, V.-H. Phan, T.-T. Le, B. T. Pham, A Novel Hybrid Model Based on a Feedforward Neural Network and One Step Secant Algorithm for Prediction of Load-Bearing Capacity of Rectangular Concrete-Filled Steel Tube Columns, Molecules, 25 (2020) 3486. https://doi.org/10.3390/molecules25153486
[34] H.-B. Ly, L. M. Le, H. T. Duong, T. C. Nguyen, T. A. Pham, T.-T. Le, V. M. Le, L. Nguyen-Ngoc, B. T. Pham, Hybrid artificial intelligence approaches for predicting critical buckling load of structural members under compression considering the influence of initial geometric imperfections, Applied Sciences, 9 (2019) 2258. https://doi.org/10.3390/app9112258
[35] D.V. Dao, S. H. Trinh, H.-B. Ly, B. T. Pham, Prediction of compressive strength of geopolymer concrete using entirely steel slag aggregates: Novel hybrid artificial intelligence approaches, Applied Sciences, 9 (2019) 1113. https://doi.org/10.3390/app9061113
[36] D. V. Dao, H. Adeli, H.-B. Ly, L. M. Le, V. M. Le, T.-T. Le, B. T. Pham, A sensitivity and robustness analysis of GPR and ANN for high-performance concrete compressive strength prediction using a Monte Carlo simulation, Sustainability, 12 (2020) 830. https://doi.org/10.3390/su12030830
[37] K. G. Sheela, S. N. Deepa, Review on methods to fix number of hidden neurons in neural networks, Mathematical Problems in Engineering, 2013 (2013). https://doi.org/10.1155/2013/425740
[38] P. B. Cachim, Using artificial neural networks for calculation of temperatures in timber under fire loading, Construction and Building Materials, 25 (2011) 4175-4180. https://doi.org/10.1016/j.conbuildmat.2011.04.054
[39] G. Cybenko, Approximation by superpositions of a sigmoidal function, Mathematics of Control, Signals and Systems, 2 (1989) 303–314
[40] D. G. Bounds, P. J. Lloyd, B. G. Mathew, G. Waddell, A multilayer perceptron network for the diagnosis of low back pain., in: ICNN, (1988) 481-489. https://doi.org/10.1109/ICNN.1988.23963
[41] S. Nagendra, Practical Aspects of Using Neural Networks: Necessary Preliminary Specifications, Technical Paper, GE Research and Development Center, (1998).
[42] K. P. George, Prediction of resilient modulus from soil index properties, University of Mississippi, (2004). https://rosap.ntl.bts.gov/view/dot/16187
Tải xuống
Chưa có dữ liệu thống kê
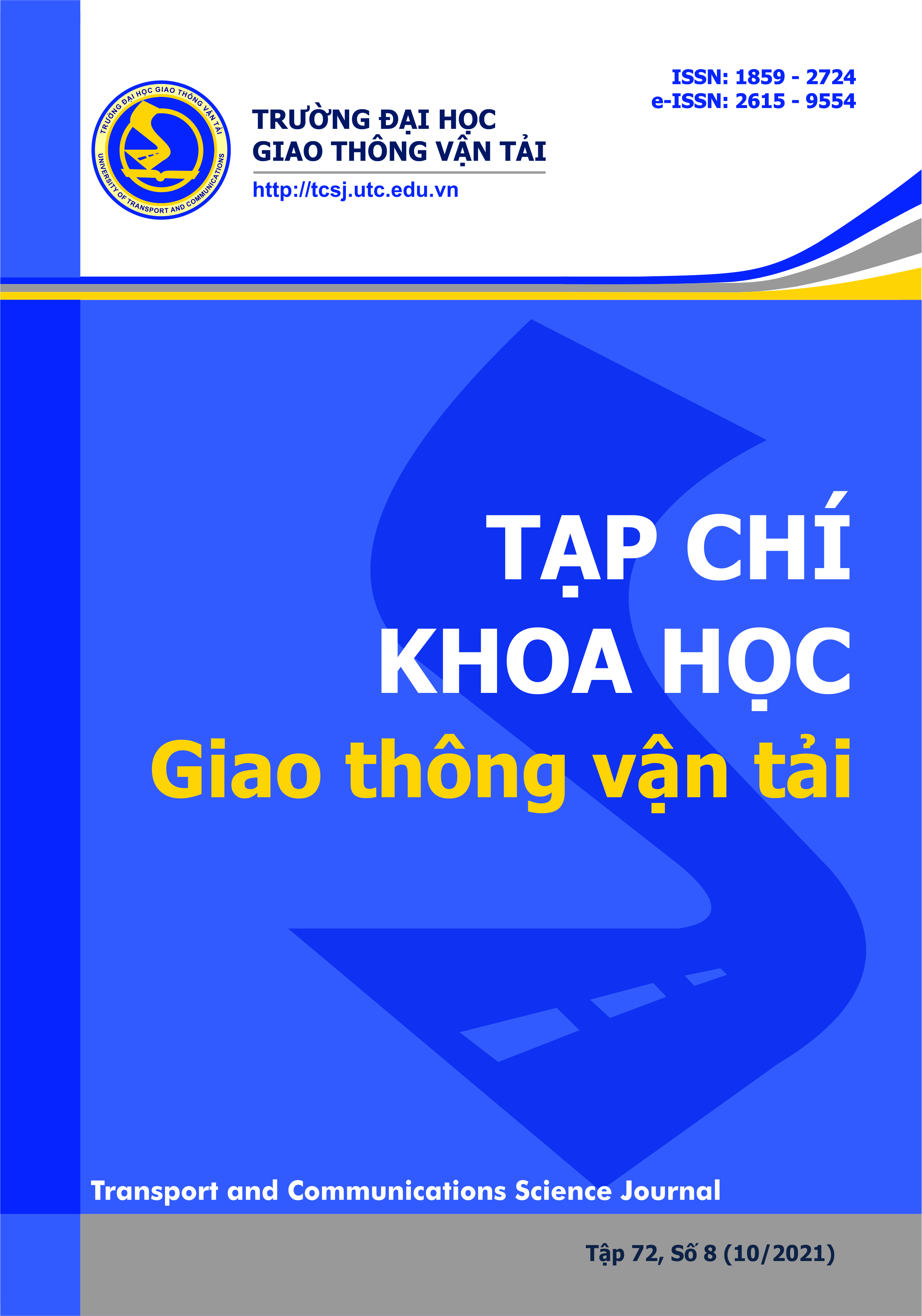
Nhận bài
05/04/2021
Nhận bài sửa
17/06/2021
Chấp nhận đăng
30/06/2021
Xuất bản
15/10/2021
Chuyên mục
Công trình khoa học
Kiểu trích dẫn
Lý Hải, B., Nguyễn Thùy, A., Mai Thị Hải, V., & Nguyễn Hữu, H. (1634230800). Nghiên cứu ứng dụng mô hình mạng nơ ron nhân tạo dự báo mô đun đàn hồi động của vật liệu đất đắp nền đường. Tạp Chí Khoa Học Giao Thông Vận Tải, 72(8), 994-1009. https://doi.org/10.47869/tcsj.72.8.12
Số lần xem tóm tắt
371
Số lần xem bài báo
687