Fuzzy-based quantification of congestion for traffic control
Email:
Trinhdinhtoan@tlu.edu.vn
Từ khóa:
Fuzzy Set; Fuzzy Rule; Congestion Level; Traffic State.
Tóm tắt
This paper presents a methodology for appraisal of congestion level for traffic control on expressways using fuzzy logic. The congestion level indicates the severity of congestion and is estimated using speed and density, being the basic traffic parameters that describe state of a traffic stream. Formulation of the fuzzy rule base is made based on knowledge on traffic flow theory and engineering judgments. Field data on a segment of the Pan-Island Expressway of Singapore were used to estimate the congestion levels for three scenarios: single input variable (speed or density) and combined input variables (speed and density), represented by congestion level on a [0 1] scale. The results showed that there were big gaps between the congestion levels evaluated based specifically on speed and density alone (single state variable), and the congestion levels estimated from both variables lie in between. Given the uncertainty in traffic data collection and dynamic nature of traffic flow, this indicates that it may be inadequate to evaluate traffic congestion level using a single variable, and the use of both speed and density represent the state of a traffic stream more properly. The study results also show that the fuzzy logic approach provides flexible combination of state variables to obtain the congestion level and to describe gradual transition of traffic state, which is particularly important under the heavy congested conditions.Tài liệu tham khảo
[1]. Highway Capacity Manual, Sixth Edition: A Guide for Multimodal Mobility Analysis, Washington, D.C. (2020).
[2]. A. M. Rao, K. R. Rao, Measuring Urban Traffic Congestion - a Review, International Journal for Traffic and Transport Engineering, 2 (2012) 286-305. https://doi.org/10.7708/ijtte.2012.2(4).01
[3]. W-X. Wang, R-J. Guo, J. Yu, Research on Road Traffic Congestion level Based on Comprehensive Parameters: Taking Dalian City as an Example, Advances in Mechanical Engineering, 10 (2018) 1-8. https://doi.org/10.1177/1687814018781482
[4]. L. A. Zadeh, Fuzzy Logic, Neural Networks, and Soft Computing. Communication of the ACM, 37 (1994) 77. https://doi.org/10.1145/175247.175255
[5]. K. Hamad, S. Kikuchi, Developing a Measure of Traffic Congestion - Fuzzy Inference Approach, Transportation Research Record: Journal of the Transportation Research Board, 1802 (2002) 77-85. https://doi.org/10.3141/1802-10
[6]. M. Arabani, S. Pourzeynali, Fuzzy Logic Methodology to Evaluate the Service Level of Freeways Basic Segments, Iranian Journal of Science & Technology Transaction B: Engineering, 29 (2005) 281-288. https://citeseerx.ist.psu.edu/viewdoc/download?doi=10.1.1.515.4924&rep=rep1&type=pdf
[7]. M. D. Munajat, D. Widyantoro, R. Munir, A New Method for Analyzing Congestion Levels Based on Road Density and Vehicle Speed, Journal of Theoretical and Applied Information Technology, 95 (2017) 6454-6471. http://www.jatit.org/volumes/Vol95No23/10Vol95No23.pdf
[8]. Bertini, R. Lawrence, You Are the Traffic Jam: An Examination of Congestion Measures, Presented at 85th Annual Meeting of the Transportation Research Board, Washington, D.C., (2006) 1419.
[9]. D. C. Xiao, S. Yun, An Improved Non-Linear Fuzzy Comprehensive Method for Assessing Urban Freeway Network Traffic Congestion Level, Open Journal of Transportation Technologies, 9 (2020) 68-78. https://doi.org/10.12677/OJTT.2020.92009
[10]. F. He et al., A Traffic Congestion Assessment Method for Urban Road Networks Based on Speed Performance Index, Procedia Engineering, 137 (2016) 425-433. https://doi.org/10.1016/j.proeng.2016.01.277
[2]. A. M. Rao, K. R. Rao, Measuring Urban Traffic Congestion - a Review, International Journal for Traffic and Transport Engineering, 2 (2012) 286-305. https://doi.org/10.7708/ijtte.2012.2(4).01
[3]. W-X. Wang, R-J. Guo, J. Yu, Research on Road Traffic Congestion level Based on Comprehensive Parameters: Taking Dalian City as an Example, Advances in Mechanical Engineering, 10 (2018) 1-8. https://doi.org/10.1177/1687814018781482
[4]. L. A. Zadeh, Fuzzy Logic, Neural Networks, and Soft Computing. Communication of the ACM, 37 (1994) 77. https://doi.org/10.1145/175247.175255
[5]. K. Hamad, S. Kikuchi, Developing a Measure of Traffic Congestion - Fuzzy Inference Approach, Transportation Research Record: Journal of the Transportation Research Board, 1802 (2002) 77-85. https://doi.org/10.3141/1802-10
[6]. M. Arabani, S. Pourzeynali, Fuzzy Logic Methodology to Evaluate the Service Level of Freeways Basic Segments, Iranian Journal of Science & Technology Transaction B: Engineering, 29 (2005) 281-288. https://citeseerx.ist.psu.edu/viewdoc/download?doi=10.1.1.515.4924&rep=rep1&type=pdf
[7]. M. D. Munajat, D. Widyantoro, R. Munir, A New Method for Analyzing Congestion Levels Based on Road Density and Vehicle Speed, Journal of Theoretical and Applied Information Technology, 95 (2017) 6454-6471. http://www.jatit.org/volumes/Vol95No23/10Vol95No23.pdf
[8]. Bertini, R. Lawrence, You Are the Traffic Jam: An Examination of Congestion Measures, Presented at 85th Annual Meeting of the Transportation Research Board, Washington, D.C., (2006) 1419.
[9]. D. C. Xiao, S. Yun, An Improved Non-Linear Fuzzy Comprehensive Method for Assessing Urban Freeway Network Traffic Congestion Level, Open Journal of Transportation Technologies, 9 (2020) 68-78. https://doi.org/10.12677/OJTT.2020.92009
[10]. F. He et al., A Traffic Congestion Assessment Method for Urban Road Networks Based on Speed Performance Index, Procedia Engineering, 137 (2016) 425-433. https://doi.org/10.1016/j.proeng.2016.01.277
Tải xuống
Chưa có dữ liệu thống kê
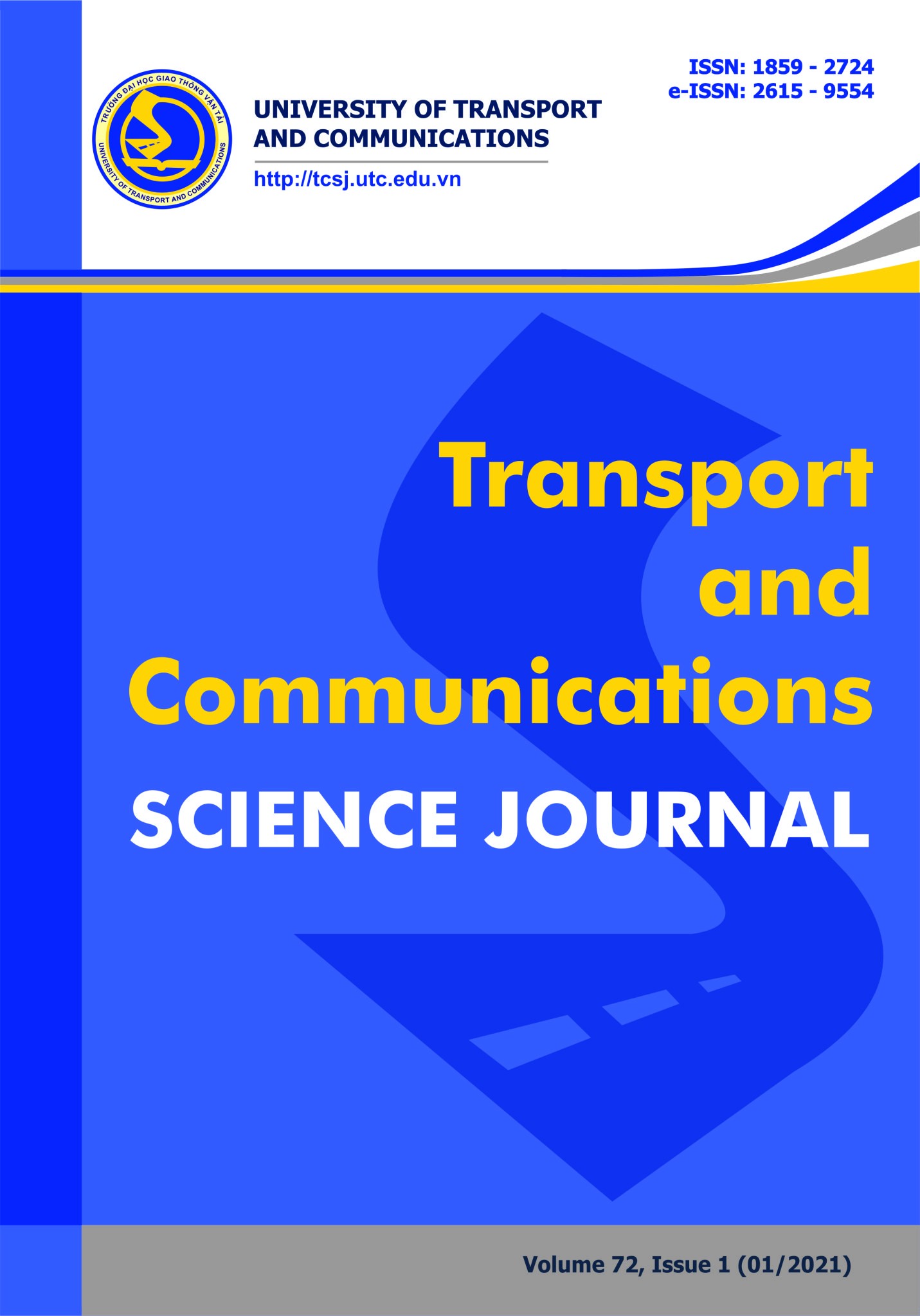
Nhận bài
05/10/2020
Nhận bài sửa
30/10/2020
Chấp nhận đăng
06/11/2020
Xuất bản
25/01/2021
Chuyên mục
Công trình khoa học
Kiểu trích dẫn
Toan Trinh, D. (7600). Fuzzy-based quantification of congestion for traffic control. Tạp Chí Khoa Học Giao Thông Vận Tải, 72(1), 1-8. https://doi.org/10.47869/tcsj.72.1.1
Số lần xem tóm tắt
189
Số lần xem bài báo
173