Phân loại hư hỏng khung thép bằng mạng nơ ron tích chập một chiều và cơ chế chú ý kênh
Email:
ngochoa@utc.edu.vn
Từ khóa:
phân loại hư hỏng, dữ liệu thời gian, mạng tích chập một chiều, cơ chế chú ý kênh
Tóm tắt
Trong lĩnh vực giám sát sức khỏe kết cấu (SHM), việc nghiên cứu các mô hình học sâu như mạng nơ-ron tích chập một chiều (1DCNN) để xử lý, phân tích các bộ dữ liệu thời gian đã được đặc biệt quan tâm. Tuy nhiên, mô hình 1DCNN không đạt được độ chính xác cao với dữ liệu có chiều dài quá lớn. Do đó, nghiên cứu này đề xuất phân loại chính xác hư hỏng trong kết cấu khung thép bằng cách kết hợp 1DCNN với cơ chế chú ý kênh (CA). Sự kết hợp này cải thiện hiệu năng của mô hình trong việc tập trung vào các đặc trưng nổi bật trong dữ liệu chuỗi thời gian, từ đó nâng cao độ chính xác trong phân loại. Nghiên cứu áp dụng bộ dữ liệu Qatar University Grandstand Simulator (QUGS), một bộ dữ liệu quy mô lớn được xây dựng cho mục đích kiểm tra hiệu quả các mô hình trong lĩnh vực SHM. Thông qua quá trình huấn luyện, mô hình đề xuất 1DCNN-CA đạt hiệu suất vượt trội so với mô hình 1DCNN truyền thống, với độ chính xác cao hơn trong cả hai tập dữ liệu xác thực và kiểm tra lần lượt là 95,7% và 93,6%. Các kết quả thu được cho thấy việc tích hợp cơ chế chú ý kênh cải thiện đáng kể độ chính xác của các mô hình học sâu trong bài toán phân loại hư hỏng và có nhiều tiềm năng trong các vấn đề thuộc lĩnh vực SHMTài liệu tham khảo
[1]. Phạm Hồng Quân, Hồ Xuân Nam, Mai Đức Anh, Nguyễn Thị Cẩm Nhung, Chẩn đoán hư hỏng cầu giàn thép sử dụng mạng hồi quy cải tiến dựa trên mô hình số được cập nhật từ dữ liệu thu được từ cảm biến quang, Tạp chí Khoa học Giao thông vận tải, 75 (2024) 2000–2014. https://doi.org/10.47869/tcsj.75.6.6
[2]. T. Bui Tien, T. Vu Quang, L. Nguyen Ngoc, H. Tran Ngoc, Time series data recovery in SHM of large-scale bridges: Leveraging GAN and Bi-LSTM networks, Structures, 63 (2024) 106368. https://doi.org/10.1016/j.istruc.2024.106368
[3]. T. Le-Xuan, T. Bui-Tien, H. Tran-Ngoc, A novel approach model design for signal data using 1DCNN combing with LSTM and ResNet for damaged detection problem, Structures, 59 (2024) 105784. https://doi.org/10.1016/j.istruc.2023.105784
[4]. O. Avci, O. Abdeljaber, S. Kiranyaz, M. Hussein, D.J. Inman, Wireless and real-time structural damage detection: A novel decentralized method for wireless sensor networks, Journal of Sound and Vibration, 424 (2018) 158–172. https://doi.org/10.1016/j.jsv.2018.03.008
[5]. O. Avci, O. Abdeljaber, S. Kiranyaz, D. Inman, Structural Damage Detection in Real Time: Implementation of 1D Convolutional Neural Networks for SHM Applications, In Proceedings of the 35th IMAC, A Conference and Exposition on Structural Dynamics, 2017 (2017) 49-54. https://doi.org/10.1007/978-3-319-54109-9
[6]. S. Kiranyaz, T. Ince, O. Abdeljaber, O. Avci, M. Gabbouj, 1-D Convolutional Neural Networks for Signal Processing Applications, ICASSP 2019 - 2019 IEEE International Conference on Acoustics, Speech and Signal Processing (ICASSP), 12-17 (2019) 8360-8364. https://doi.org/10.1109/ICASSP.2019.8682194
[7]. O. Abdeljaber, O. Avci, M.S. Kiranyaz, B. Boashash, H. Sodano, D.J. Inman, 1-D CNNs for structural damage detection: Verification on a structural health monitoring benchmark data, Neurocomputing, 275 (2018) 1308–1317. https://doi.org/10.1016/j.neucom.2017.09.069
[8]. O. Avci, O. Abdeljaber, S. Kiranyaz, D. Inman, Convolutional Neural Networks for Real-Time and Wireless Damage Detection, Shamim Pakzad, Proceedings of the 37th IMAC, A Conference and Exposition on Structural Dynamics, 2019 (2019) 129-136. https://doi.org/10.1007/978-3-030-12115-0_17
[9]. H. Fathnejat, B. Ahmadi-Nedushan, S. Hosseininejad, M. Noori, W.A. Altabey, A data-driven structural damage identification approach using deep convolutional-attention-recurrent neural architecture under temperature variations, Engineering Structures, 276 (2023) 115311. https://doi.org/10.1016/j.engstruct.2022.115311
[10]. H. Tran-Ngoc, Q. Nguyen-Huu, T. Nguyen-Chi, T. Bui-Tien, Enhancing damage detection in truss bridges through structural stiffness reduction using 1DCNN, BiLSTM, and data augmentation techniques, Structures 68 (2024) 107035. https://doi.org/10.1016/j.istruc.2024.107035
[11]. T. Bui-Tien, T. Nguyen-Chi, T. Le-Xuan, H. Tran-Ngoc, Enhancing bridge damage assessment: Adaptive cell and deep learning approaches in time-series analysis, Construction and Building Materials, 439 (2024) 137240. https://doi.org/10.1016/j.conbuildmat.2024.137240
[12]. Z. Niu, G. Zhong, H. Yu, A review on the attention mechanism of deep learning, Neurocomputing, 452 (2021) 48–62. https://doi.org/10.1016/j.neucom.2021.03.091
[13]. X. Zhao, M. Bai, X. Yang, J. Liu, D. Yu, J. Chang, Short-term probabilistic predictions of wind multi-parameter based on one-dimensional convolutional neural network with attention mechanism and multivariate copula distribution estimation, Energy, 234 (2021) 121306. https://doi.org/10.1016/j.energy.2021.121306
[14]. K. Wang, C. Ma, Y. Qiao, X. Lu, W. Hao, S. Dong, A hybrid deep learning model with 1DCNN-LSTM-Attention networks for short-term traffic flow prediction, Physica A: Statistical Mechanics and Its Applications, 583 (2021) 126293. https://doi.org/10.1016/j.physa.2021.126293.
[15]. H. Hu, Y. Mei, Y. Wei, Z. Xu, Y. Zhao, H. Xu, X. Mao, L. Huang, Chemical composition prediction in goji (Lycium barbarum) using hyperspectral imaging and multi-task 1DCNN with attention mechanism, LWT, 204 (2024) 116436. https://doi.org/10.1016/j.lwt.2024.116436.
[16]. X. Zhang, C. He, Y. Lu, B. Chen, L. Zhu, L. Zhang, Fault diagnosis for small samples based on attention mechanism, Measurement, 187 (2022) 110242. https://doi.org/10.1016/j.measurement.2021.110242
[17]. T.-T. Vo, M.-K. Liu, M.-Q. Tran, Harnessing attention mechanisms in a comprehensive deep learning approach for induction motor fault diagnosis using raw electrical signals, Engineering Applications of Artificial Intelligence, 129 (2024) 107643. https://doi.org/10.1016/j.engappai.2023.107643
[18]. S. Zhang, W. Liang, W. Zhao, Z. Luan, C. Wang, K. Xu, Electro-hydraulic SBW fault diagnosis method based on novel 1DCNN-LSTM with attention mechanisms and transfer learning, Mechanical Systems and Signal Processing, 220 (2024) 111644. https://doi.org/10.1016/j.ymssp.2024.111644
[19]. Structural Dynamics Team, A New Experimental Benchmark Problem for Vibration-Based Structural Health Monitoring (SHM). https://www.structuralvibration.com/benchmark/, 2018, truy cập ngày 20 tháng 08 năm 2024.
[20]. O. Abdeljaber, O. Avci, S. Kiranyaz, M. Gabbouj, D.J. Inman, Real-time vibration-based structural damage detection using one-dimensional convolutional neural networks, Journal of Sound and Vibration, 388 (2017) 154–170. https://doi.org/10.1016/j.jsv.2016.10.043
[2]. T. Bui Tien, T. Vu Quang, L. Nguyen Ngoc, H. Tran Ngoc, Time series data recovery in SHM of large-scale bridges: Leveraging GAN and Bi-LSTM networks, Structures, 63 (2024) 106368. https://doi.org/10.1016/j.istruc.2024.106368
[3]. T. Le-Xuan, T. Bui-Tien, H. Tran-Ngoc, A novel approach model design for signal data using 1DCNN combing with LSTM and ResNet for damaged detection problem, Structures, 59 (2024) 105784. https://doi.org/10.1016/j.istruc.2023.105784
[4]. O. Avci, O. Abdeljaber, S. Kiranyaz, M. Hussein, D.J. Inman, Wireless and real-time structural damage detection: A novel decentralized method for wireless sensor networks, Journal of Sound and Vibration, 424 (2018) 158–172. https://doi.org/10.1016/j.jsv.2018.03.008
[5]. O. Avci, O. Abdeljaber, S. Kiranyaz, D. Inman, Structural Damage Detection in Real Time: Implementation of 1D Convolutional Neural Networks for SHM Applications, In Proceedings of the 35th IMAC, A Conference and Exposition on Structural Dynamics, 2017 (2017) 49-54. https://doi.org/10.1007/978-3-319-54109-9
[6]. S. Kiranyaz, T. Ince, O. Abdeljaber, O. Avci, M. Gabbouj, 1-D Convolutional Neural Networks for Signal Processing Applications, ICASSP 2019 - 2019 IEEE International Conference on Acoustics, Speech and Signal Processing (ICASSP), 12-17 (2019) 8360-8364. https://doi.org/10.1109/ICASSP.2019.8682194
[7]. O. Abdeljaber, O. Avci, M.S. Kiranyaz, B. Boashash, H. Sodano, D.J. Inman, 1-D CNNs for structural damage detection: Verification on a structural health monitoring benchmark data, Neurocomputing, 275 (2018) 1308–1317. https://doi.org/10.1016/j.neucom.2017.09.069
[8]. O. Avci, O. Abdeljaber, S. Kiranyaz, D. Inman, Convolutional Neural Networks for Real-Time and Wireless Damage Detection, Shamim Pakzad, Proceedings of the 37th IMAC, A Conference and Exposition on Structural Dynamics, 2019 (2019) 129-136. https://doi.org/10.1007/978-3-030-12115-0_17
[9]. H. Fathnejat, B. Ahmadi-Nedushan, S. Hosseininejad, M. Noori, W.A. Altabey, A data-driven structural damage identification approach using deep convolutional-attention-recurrent neural architecture under temperature variations, Engineering Structures, 276 (2023) 115311. https://doi.org/10.1016/j.engstruct.2022.115311
[10]. H. Tran-Ngoc, Q. Nguyen-Huu, T. Nguyen-Chi, T. Bui-Tien, Enhancing damage detection in truss bridges through structural stiffness reduction using 1DCNN, BiLSTM, and data augmentation techniques, Structures 68 (2024) 107035. https://doi.org/10.1016/j.istruc.2024.107035
[11]. T. Bui-Tien, T. Nguyen-Chi, T. Le-Xuan, H. Tran-Ngoc, Enhancing bridge damage assessment: Adaptive cell and deep learning approaches in time-series analysis, Construction and Building Materials, 439 (2024) 137240. https://doi.org/10.1016/j.conbuildmat.2024.137240
[12]. Z. Niu, G. Zhong, H. Yu, A review on the attention mechanism of deep learning, Neurocomputing, 452 (2021) 48–62. https://doi.org/10.1016/j.neucom.2021.03.091
[13]. X. Zhao, M. Bai, X. Yang, J. Liu, D. Yu, J. Chang, Short-term probabilistic predictions of wind multi-parameter based on one-dimensional convolutional neural network with attention mechanism and multivariate copula distribution estimation, Energy, 234 (2021) 121306. https://doi.org/10.1016/j.energy.2021.121306
[14]. K. Wang, C. Ma, Y. Qiao, X. Lu, W. Hao, S. Dong, A hybrid deep learning model with 1DCNN-LSTM-Attention networks for short-term traffic flow prediction, Physica A: Statistical Mechanics and Its Applications, 583 (2021) 126293. https://doi.org/10.1016/j.physa.2021.126293.
[15]. H. Hu, Y. Mei, Y. Wei, Z. Xu, Y. Zhao, H. Xu, X. Mao, L. Huang, Chemical composition prediction in goji (Lycium barbarum) using hyperspectral imaging and multi-task 1DCNN with attention mechanism, LWT, 204 (2024) 116436. https://doi.org/10.1016/j.lwt.2024.116436.
[16]. X. Zhang, C. He, Y. Lu, B. Chen, L. Zhu, L. Zhang, Fault diagnosis for small samples based on attention mechanism, Measurement, 187 (2022) 110242. https://doi.org/10.1016/j.measurement.2021.110242
[17]. T.-T. Vo, M.-K. Liu, M.-Q. Tran, Harnessing attention mechanisms in a comprehensive deep learning approach for induction motor fault diagnosis using raw electrical signals, Engineering Applications of Artificial Intelligence, 129 (2024) 107643. https://doi.org/10.1016/j.engappai.2023.107643
[18]. S. Zhang, W. Liang, W. Zhao, Z. Luan, C. Wang, K. Xu, Electro-hydraulic SBW fault diagnosis method based on novel 1DCNN-LSTM with attention mechanisms and transfer learning, Mechanical Systems and Signal Processing, 220 (2024) 111644. https://doi.org/10.1016/j.ymssp.2024.111644
[19]. Structural Dynamics Team, A New Experimental Benchmark Problem for Vibration-Based Structural Health Monitoring (SHM). https://www.structuralvibration.com/benchmark/, 2018, truy cập ngày 20 tháng 08 năm 2024.
[20]. O. Abdeljaber, O. Avci, S. Kiranyaz, M. Gabbouj, D.J. Inman, Real-time vibration-based structural damage detection using one-dimensional convolutional neural networks, Journal of Sound and Vibration, 388 (2017) 154–170. https://doi.org/10.1016/j.jsv.2016.10.043
Tải xuống
Chưa có dữ liệu thống kê
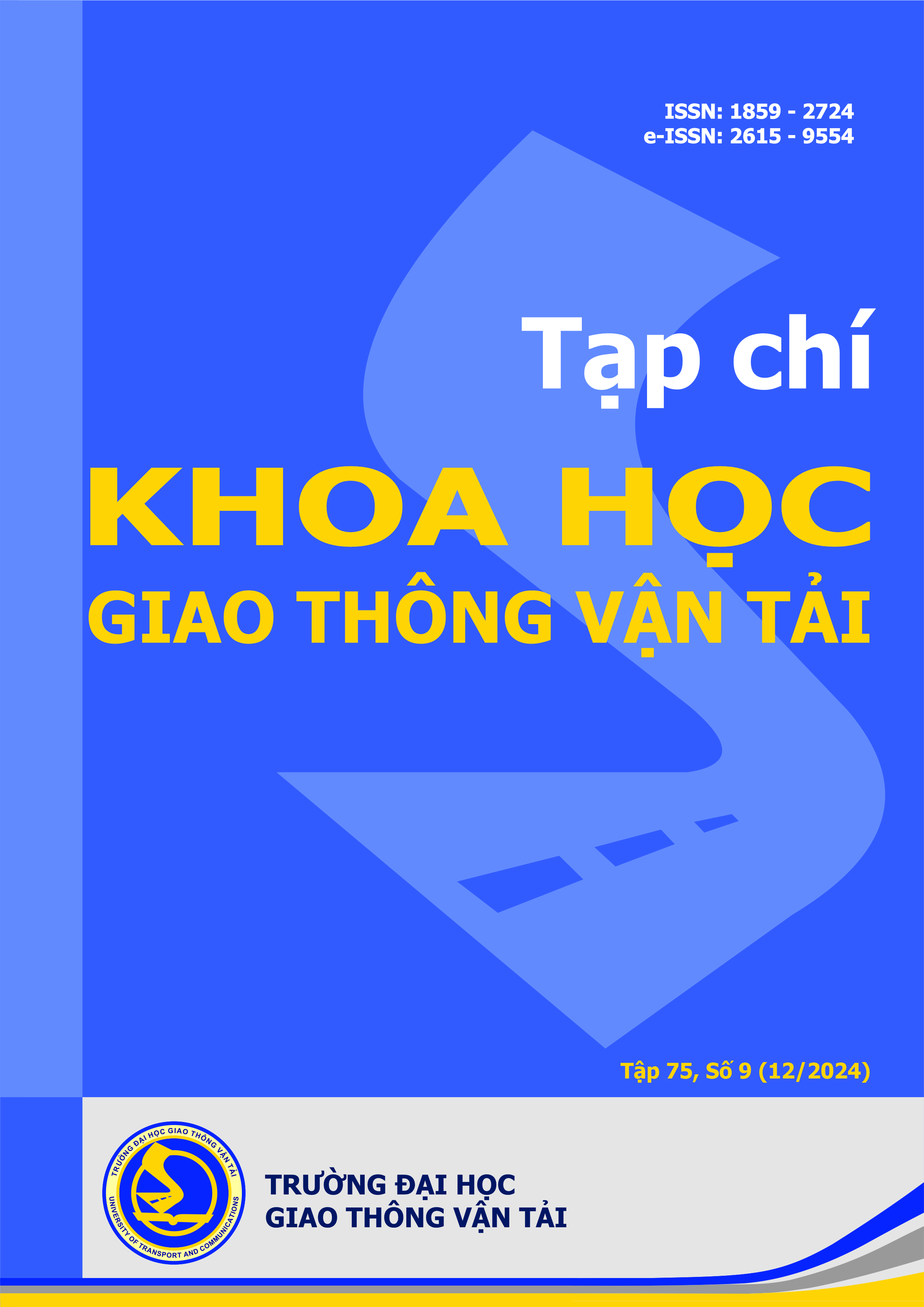
Nhận bài
17/09/2024
Nhận bài sửa
02/11/2024
Chấp nhận đăng
10/12/2024
Xuất bản
15/12/2024
Chuyên mục
Công trình khoa học
Kiểu trích dẫn
Nguyễn Hữu, Q., Lê Văn, V., & Trần Ngọc, H. (1734195600). Phân loại hư hỏng khung thép bằng mạng nơ ron tích chập một chiều và cơ chế chú ý kênh. Tạp Chí Khoa Học Giao Thông Vận Tải, 75(9), 2333-2344. https://doi.org/10.47869/tcsj.75.9.8
Số lần xem tóm tắt
118
Số lần xem bài báo
53