Convolutional neural network for determining the flow field around an airfoil and blunt-based models
Email:
tranthehung_k24@lqdtu.edu.vn
Tóm tắt
The convolutional neural network is widely applied in the classification of images and medicine. Some current networks are used in aerospace engineering and show a high potential in determining aerodynamic forces and flow fields. This article constructs a convolutional neural network for predicting pressure and velocity fields around a two-dimensional aircraft wing model (airfoil model). Training data is computed using the Reynolds-averaged method, and then extracted, focusing on the flow around the wing. Input data includes geometric parameters, and airfoil inlet velocity, and output data includes pressure field and flow velocity around the airfoil. The convolutional neural network is based on improving the U-Net network model, commonly used in medical applications. The results show that the convolutional neural network accurately predicts flow around the airfoil, with an average error below 3%. Therefore, this network can be used and further developed to predict flow around the wing. The network is then applied to predict the pressure and pressure fields around a blunt-based model with different aspect ratios. The main feature of the flow can be extracted from the network. Results related to pressure distribution, velocity, and method error are presented and discussed in the study. This study also suggests improving the network and applying it to pressure and velocity fields in aerospace engineeringTài liệu tham khảo
[1]. M. Drela, XFOIL: An analysis and design system for low Reynolds number airfoils, in Low Reynolds Number Aerodynamics, in Proceedings of the Conference Notre Dame, Indiana, USA, 5–7 (1989) 1–12. https://doi.org/10.1007/978-3-642-84010-4_1
[2]. T.H. Tran, D.A. Le, T.M. Nguyen, C.T. Dao, V.Q. Duong, Comparison of numerical and experimental methods in determining boundary layer of axisymmetric model, in International Conference on Advanced Mechanical Engineering, Automation and Sustainable Development, (2022) 297–302. https://doi.org/10.1007/978-3-030-99666-6_45
[3]. D.Q. Nguyen, T.H. Tran, D.A. Le, N.T. Hieu, V.K. Pham, T.S. Ha, Drag reduction for axisymmetric boattail model by longitudinal groove cavity under low-speed conditions, Journal of Mechanical Science and Technology, 38 (8) (2024) 4209–4220. http://doi.org/10.1007/s12206-024-0718-4
[4]. G. Du, X. Cao, J. Liang, X. Chen, Y. Zhan, Medical image segmentation based on u-net: A review, Journal of Imaging Science Technology, 64 (2020). http://doi.org/10.2352/J.ImagingSci.Technol.2020.64.2.020508
[5]. H.T. Tran, K.V. Pham, A.D. Le, C.T. Dinh, Frequency characteristics of axisymmetric conical boattail models with different slant angles, Physics of Fluids, 35 (2023) 095113. https://doi.org/10.1063/5.0160053
[6]. N. Thuerey, K. Weibenow, L. Prantl, X. Hu, Deep learning methods for Reynolds-averaged Navier–Stokes simulations of airfoil flows, AIAA Journal, 58 (2020) 25–36. http://doi.org/ 10.2514/1.j058291
[7]. N. Siddique, S. Paheding, C.P. Elkin, V. Devabhaktuni, U-net and its variants for medical image segmentation: A review of theory and applications. IEEE Access, 9 (2021) 82031-82057. http://doi.org/10.1109/ACCESS.2021.3086020
[8]. X. Wu, D. Hong, J. Chanussot, UIU-Net: U-Net in U-Net for infrared small object detection. IEEE Transactions on Image Processing, 32 (2022) 364-376. http://doi.org/10.1109/TIP.2022.3228497
[9]. P. Spalart, S. Allmaras, A one-equation turbulence model for aerodynamic flows. 30th aerospace sciences meeting and exhibit, 439 (1992). https://doi.org/10.2514/6.1992-439
[10]. C. Kostić, Review of the Spalart-Allmaras turbulence model and its modifications to three-dimensional supersonic configurations, Scientific Technical Review, 65 (2015) 43-49.
[11]. K.V. Pham, H.T. Tran, D.T. Nguyen, A.D. Le, Q.D. Nguyen, D.T. Pham, Delayed detached eddy simulation for wake flow analysis of axisymmetric boattail models under low-speed conditions, Physics of Fluids, 36 (2024) 035159. https://doi.org/10.1063/5.0188363
[12]. T.H. Tran, The effect of boattail angles on the near-wake structure of axisymmetric afterbody models at low-speed condition, International Journal of Aerospace Engineering, (2020) 1-14. https://doi.org/10.1155/2020/7580174
[13]. T. Nonomura, K. Sato, K. Fukata, H. Nagaike, H. Okuizumi, Y. Konishi, H. Sawada, Effect of fineness ratios of 0.75–2.0 on aerodynamic drag of freestream-aligned circular cylinders measured using a magnetic suspension and balance system, Experiments in Fluids, 59 (2018) 1-12. https://doi.org/10.1007/s00348-018-2531-2
[2]. T.H. Tran, D.A. Le, T.M. Nguyen, C.T. Dao, V.Q. Duong, Comparison of numerical and experimental methods in determining boundary layer of axisymmetric model, in International Conference on Advanced Mechanical Engineering, Automation and Sustainable Development, (2022) 297–302. https://doi.org/10.1007/978-3-030-99666-6_45
[3]. D.Q. Nguyen, T.H. Tran, D.A. Le, N.T. Hieu, V.K. Pham, T.S. Ha, Drag reduction for axisymmetric boattail model by longitudinal groove cavity under low-speed conditions, Journal of Mechanical Science and Technology, 38 (8) (2024) 4209–4220. http://doi.org/10.1007/s12206-024-0718-4
[4]. G. Du, X. Cao, J. Liang, X. Chen, Y. Zhan, Medical image segmentation based on u-net: A review, Journal of Imaging Science Technology, 64 (2020). http://doi.org/10.2352/J.ImagingSci.Technol.2020.64.2.020508
[5]. H.T. Tran, K.V. Pham, A.D. Le, C.T. Dinh, Frequency characteristics of axisymmetric conical boattail models with different slant angles, Physics of Fluids, 35 (2023) 095113. https://doi.org/10.1063/5.0160053
[6]. N. Thuerey, K. Weibenow, L. Prantl, X. Hu, Deep learning methods for Reynolds-averaged Navier–Stokes simulations of airfoil flows, AIAA Journal, 58 (2020) 25–36. http://doi.org/ 10.2514/1.j058291
[7]. N. Siddique, S. Paheding, C.P. Elkin, V. Devabhaktuni, U-net and its variants for medical image segmentation: A review of theory and applications. IEEE Access, 9 (2021) 82031-82057. http://doi.org/10.1109/ACCESS.2021.3086020
[8]. X. Wu, D. Hong, J. Chanussot, UIU-Net: U-Net in U-Net for infrared small object detection. IEEE Transactions on Image Processing, 32 (2022) 364-376. http://doi.org/10.1109/TIP.2022.3228497
[9]. P. Spalart, S. Allmaras, A one-equation turbulence model for aerodynamic flows. 30th aerospace sciences meeting and exhibit, 439 (1992). https://doi.org/10.2514/6.1992-439
[10]. C. Kostić, Review of the Spalart-Allmaras turbulence model and its modifications to three-dimensional supersonic configurations, Scientific Technical Review, 65 (2015) 43-49.
[11]. K.V. Pham, H.T. Tran, D.T. Nguyen, A.D. Le, Q.D. Nguyen, D.T. Pham, Delayed detached eddy simulation for wake flow analysis of axisymmetric boattail models under low-speed conditions, Physics of Fluids, 36 (2024) 035159. https://doi.org/10.1063/5.0188363
[12]. T.H. Tran, The effect of boattail angles on the near-wake structure of axisymmetric afterbody models at low-speed condition, International Journal of Aerospace Engineering, (2020) 1-14. https://doi.org/10.1155/2020/7580174
[13]. T. Nonomura, K. Sato, K. Fukata, H. Nagaike, H. Okuizumi, Y. Konishi, H. Sawada, Effect of fineness ratios of 0.75–2.0 on aerodynamic drag of freestream-aligned circular cylinders measured using a magnetic suspension and balance system, Experiments in Fluids, 59 (2018) 1-12. https://doi.org/10.1007/s00348-018-2531-2
Tải xuống
Chưa có dữ liệu thống kê
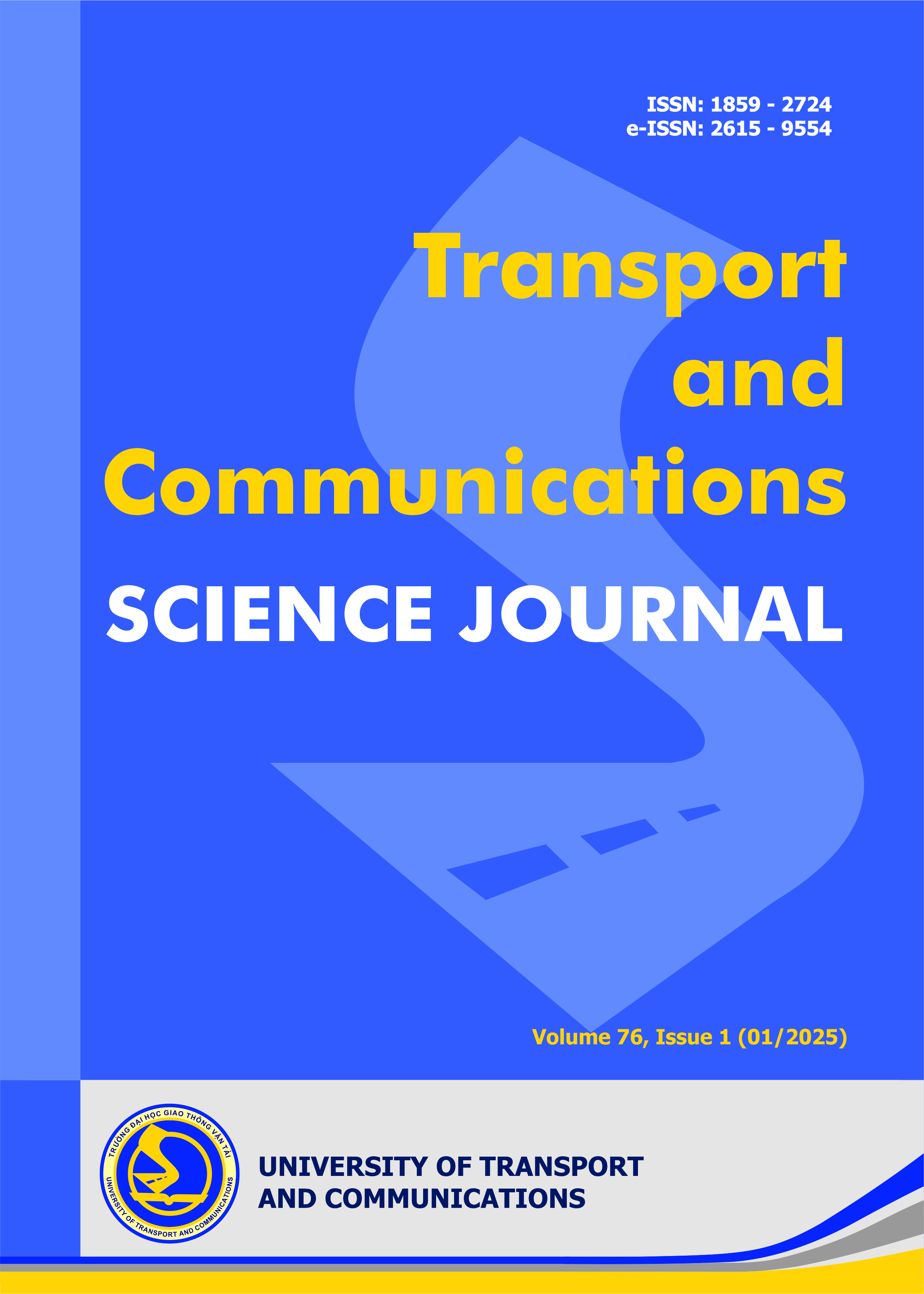
Nhận bài
05/06/2024
Nhận bài sửa
24/10/2024
Chấp nhận đăng
10/01/2025
Xuất bản
15/01/2025
Chuyên mục
Công trình khoa học
Kiểu trích dẫn
Tran The, H. (1736874000). Convolutional neural network for determining the flow field around an airfoil and blunt-based models. Tạp Chí Khoa Học Giao Thông Vận Tải, 76(1), 31-41. https://doi.org/10.47869/tcsj.76.1.3
Số lần xem tóm tắt
94
Số lần xem bài báo
31