Real-time estimation of vehicle inertia parameters based on Kalman-bucy filter
Email:
nguyentuananh@utc.edu.vn
Từ khóa:
sprung mass, moments of inertia, spatial vehicle model, real-time estimation, Kalman-Bucy filter
Tóm tắt
Vehicle inertial parameters such as mass and moments of inertia are required for most vehicle dynamic control systems. Due to the wide range variation of these parameters during vehicle operation, accurate estimation of their values in real-time plays an important role in improving the efficiency of vehicle control systems. In this article, the vehicle sprung mass and moments of inertia are estimated in real-time based on a Kalman-Bucy filter algorithm designed for a spatial vibration model of a two-axle truck. This proposed method requires measuring only the vertical, roll, and pitch velocity of the sprung mass and, therefore can reduce the sensor cost significantly. The simulation results for a random roughness road profile according to ISO 8608 class C with step variations in sprung mass and moments of inertia showed that the designed estimator rejected the process and measurement noises and tracked the real vehicle parameters effectively with acceptable errorsTài liệu tham khảo
[1]. Kanwar Bharat Singh, Saied Taheri, Integrated State and Parameter Estimation for Vehicle Dynamics Control, International Journal of Vehicle Performance, 5 (2019) 329-376.
[2]. W. Li, H. Li, K. Xu, Z. Huang, K. Li, H. Du, Estimation of Vehicle Dynamic Parameters Based on the Two-Stage Estimation Method, Sensors, 21(11) (2021) 3711. https://doi.org/10.3390/s21113711
[3]. Moustapha Doumiati, Ali Charara, Alessandro Victorino, Daniel Lechner, Vehicle Dynamics Estimation using Kalman Filtering - Experimental Validation, John Wiley & Sons, Inc., (2013).
[4]. N. Kidambi, R.L. Harne, Y. Fujii, G.M. Pietron, K.W. Wang, Methods in Vehicle Mass and Road Grade Estimation, SAE Int. J. Passeng. Cars – Mech, Syst., 7 (2014) 981-991. https://doi.org/10.4271/2014-01-0111
[5]. A. Vahidi, A. Stefanopoulou, H. Peng, Recursive Least Squares with Forgetting for Online Estimation of Vehicle Mass and Road Grade: Theory and Experiments, Vehicle System Dynamics, 43 (2005) 31-55. https://doi.org/10.1080/00423110412331290446
[6]. Daeil Kim, Seibum B. Choi, Jiwon Oh, Integrated Vehicle Mass Estimation Using Longitudinal and Roll Dynamics, The 12th International Conference on Control, Automation and Systems, Korea, (2012) 862-867.
[7]. Y. Zha, X. Liu, F. Ma, C. Liu, Vehicle State Estimation Based on Extended Kalman Filter and Radial Basis Function Neural Networks, International Journal of Distributed Sensor Networks, 18 (2022). https://doi.org/10.1177/15501329221102730
[8]. Nguyen Tuan-Anh, Nguyen Cong Tuan, Real-time Vehicle Inertial Parameters Estimation Based on a Simplified Half-Car Vertical Vibration Model. International Conference on Engineering Research and Applications ICERA 2018, LNNS, Springer, 63 (2019) 521-531.
[9]. Wanmin Li, Yan Wang, Yaping Zhang, Yunzi Yang, Kalman Filter Method Based Vehicle Mass Estimation for Automobile Suspension System, International Journal of Circuits, Systems and Signal Processing, 13 (2019) 344-351.
[10]. J. Kolansky, C. Sandu, Real-time Parameter Estimation Study for Inertia Properties of Ground Vehicles, The Archive of Mechanical Engineering, 60 (2013) 7-21.
[11]. Truong Manh Hung, Vu Van Tan, Designing a Kalman–Bucy State Estimator for a Full Vertical Car Model, Mathematical Problems in Engineering, 2023 (2023). https://doi.org/10.1155/2023/6941084
[12]. O. Donatus, O. Uchenna, K. K. Uka, I. B. Okafor, I. A. Amaefule, Implementation of Kalman-Bucy Filter for Continuous Time State Estimation in Simulink, International Research Journal of Advanced Engineering and Science, 3 (2017) 11-13.
[13]. Peter Múčka, Simulated Road Profiles According to ISO 8608 in Vibration Analysis, Journal of Testing and Evaluation, 46 (2018) 405-418.
[2]. W. Li, H. Li, K. Xu, Z. Huang, K. Li, H. Du, Estimation of Vehicle Dynamic Parameters Based on the Two-Stage Estimation Method, Sensors, 21(11) (2021) 3711. https://doi.org/10.3390/s21113711
[3]. Moustapha Doumiati, Ali Charara, Alessandro Victorino, Daniel Lechner, Vehicle Dynamics Estimation using Kalman Filtering - Experimental Validation, John Wiley & Sons, Inc., (2013).
[4]. N. Kidambi, R.L. Harne, Y. Fujii, G.M. Pietron, K.W. Wang, Methods in Vehicle Mass and Road Grade Estimation, SAE Int. J. Passeng. Cars – Mech, Syst., 7 (2014) 981-991. https://doi.org/10.4271/2014-01-0111
[5]. A. Vahidi, A. Stefanopoulou, H. Peng, Recursive Least Squares with Forgetting for Online Estimation of Vehicle Mass and Road Grade: Theory and Experiments, Vehicle System Dynamics, 43 (2005) 31-55. https://doi.org/10.1080/00423110412331290446
[6]. Daeil Kim, Seibum B. Choi, Jiwon Oh, Integrated Vehicle Mass Estimation Using Longitudinal and Roll Dynamics, The 12th International Conference on Control, Automation and Systems, Korea, (2012) 862-867.
[7]. Y. Zha, X. Liu, F. Ma, C. Liu, Vehicle State Estimation Based on Extended Kalman Filter and Radial Basis Function Neural Networks, International Journal of Distributed Sensor Networks, 18 (2022). https://doi.org/10.1177/15501329221102730
[8]. Nguyen Tuan-Anh, Nguyen Cong Tuan, Real-time Vehicle Inertial Parameters Estimation Based on a Simplified Half-Car Vertical Vibration Model. International Conference on Engineering Research and Applications ICERA 2018, LNNS, Springer, 63 (2019) 521-531.
[9]. Wanmin Li, Yan Wang, Yaping Zhang, Yunzi Yang, Kalman Filter Method Based Vehicle Mass Estimation for Automobile Suspension System, International Journal of Circuits, Systems and Signal Processing, 13 (2019) 344-351.
[10]. J. Kolansky, C. Sandu, Real-time Parameter Estimation Study for Inertia Properties of Ground Vehicles, The Archive of Mechanical Engineering, 60 (2013) 7-21.
[11]. Truong Manh Hung, Vu Van Tan, Designing a Kalman–Bucy State Estimator for a Full Vertical Car Model, Mathematical Problems in Engineering, 2023 (2023). https://doi.org/10.1155/2023/6941084
[12]. O. Donatus, O. Uchenna, K. K. Uka, I. B. Okafor, I. A. Amaefule, Implementation of Kalman-Bucy Filter for Continuous Time State Estimation in Simulink, International Research Journal of Advanced Engineering and Science, 3 (2017) 11-13.
[13]. Peter Múčka, Simulated Road Profiles According to ISO 8608 in Vibration Analysis, Journal of Testing and Evaluation, 46 (2018) 405-418.
Tải xuống
Chưa có dữ liệu thống kê
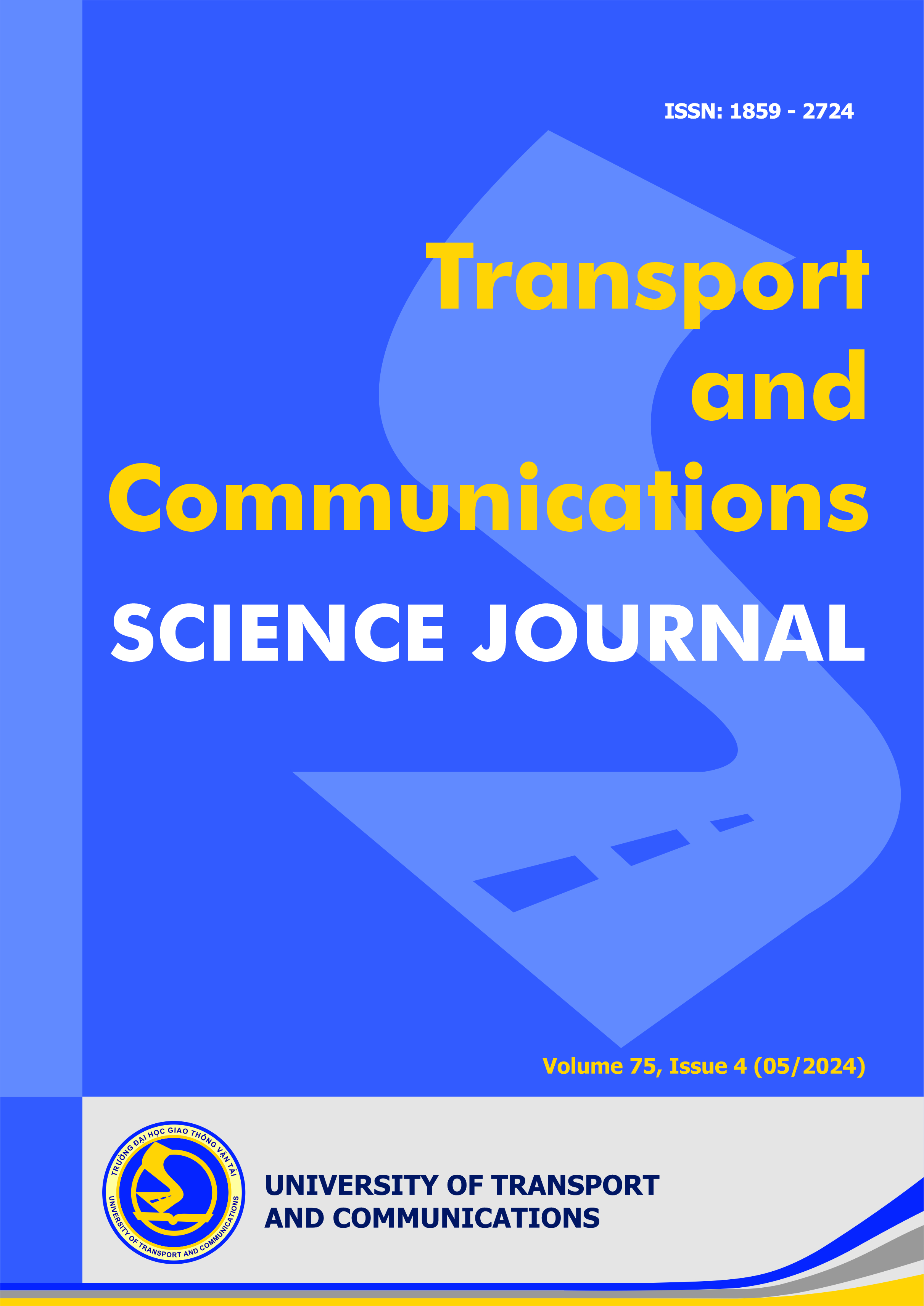
Nhận bài
13/04/2024
Nhận bài sửa
04/05/2024
Chấp nhận đăng
07/05/2024
Xuất bản
15/05/2024
Chuyên mục
Công trình khoa học
Kiểu trích dẫn
Nguyen Tuan, A. (1715706000). Real-time estimation of vehicle inertia parameters based on Kalman-bucy filter. Tạp Chí Khoa Học Giao Thông Vận Tải, 75(4), 1593-1603. https://doi.org/10.47869/tcsj.75.4.10
Số lần xem tóm tắt
160
Số lần xem bài báo
104