Dự báo ô nhiễm không khí từ dữ liệu quan trắc sử dụng các mô hình trí tuệ nhân tạo
Email:
caominhquy@utc.edu.vn
Từ khóa:
Ô nhiễm không khí, dự báo, trí tuệ nhân tạo
Tóm tắt
Ô nhiễm không khí đang tạo nên một thách thức đối với môi trường và sức khỏe của con người. Các chất gây ô nhiễm như các hạt bụi siêu nhỏ, khí độc hại phát sinh từ hoạt động của các phương tiện vận tải và quá trình sản xuất công nghiệp đang trở thành mối đe dọa lớn đối với sức khỏe con người. Không chỉ ảnh hưởng đến sức khỏe con người, ô nhiễm không khí còn góp phần vào tình trạng biến đổi khí hậu toàn cầu, gây ảnh hưởng nghiêm trọng đến sự đa dạng sinh học trên Trái Đất. Bài báo trình bày phương pháp dự báo chất lượng không khí nhằm cảnh báo sớm ô nhiễm thông qua sử dụng mô hình trí tuệ nhân tạo. Mô hình nhận dữ liệu các chất ô nhiễm từ trạm quan trắc và dự đoán nồng độ các chất ô nhiễm này trong thời gian kế tiếpTài liệu tham khảo
[1]. WHO. World Health Statistics 2021: Monitoring Health for the SDGs, Sustainable Development Goals; WHO: Geneva, Switzerland, 2021.
[2]. J. Zaheer, J. Jeon, S.B. LeeΙΙ, J.S. Kim, Effect of Particulate Matter on Human Health, Prevention, and Imaging Using PET or SPECT, Prog. Med. Phys., 29 (2018) 81-91. https://doi.org/10.14316/pmp.2018.29.3.81
[3]. Bộ TN&MT, Báo cáo Hiện trạng Môi trường Việt Nam giai đoạn 2016-2020, 2021.
[4]. Phạm Ngọc Đăng, Thực trạng chất lượng môi trường không khí Việt Nam giai đoạn 2016 – 2020, xác định các thách thức và đề xuất các giải pháp cải thiện trong 5 năm tới, Tạp chí Môi trường số tháng 1 (2022).
[5]. V. I. Kontopoulou, A. D. Athanasios, I. Kakkos, G. K. Matsopoulos., A Review of ARIMA vs. Machine Learning Approaches for Time Series Forecasting in Data Driven Networks, Future Internet, 15 (2023) 255. https://doi.org/10.3390/fi15080255
[6]. Peng Chen, Aichen Niu, Duanyang Liu, Wei Jiang, Bin Ma, Time Series Forecasting of Temperatures using SARIMA: An Example from Nanjing, IOP Conference Series: Materials Science and Engineering, 394 (2018) 052024. https://doi.org/10.1088/1757-899X/394/5/052024
[7]. Q. Song, B. S. Chissom, Forecasting enrollments with fuzzy time series – Part I. Fuzzy Sets and Systems, 54 (1993) 1–9. https://doi.org/10.1016/0165-0114(93)90355-L
[8]. C. R. Staudemeyer, E. R. Morris, Understanding LSTM -- a tutorial into Long Short-Term Memory Recurrent Neural Networks, (2019) 1-42, https://doi.org/10.48550/arXiv.1909.09586
[9]. Ahmed Tealab, Time series forecasting using artificial neural networks methodologies: A systematic review, Future Computing and Informatics Journal, 3 (2018) 334-340. https://doi.org/10.1016/j.fcij.2018.10.003
[10]. Gjylapi, Dezdemona & Proko, Eljona, Recurrent Neural Networks in Time Series Prediction, 2017.
[11]. David Kriesel, A Brief Introduction to Neural Networks, NeuralNetworks, 2007.
[12]. Hansika Hewamalage, Christoph Bergmeir, Kasun Bandara, Recurrent Neural Networks for Time Series Forecasting: Current status and future directions, International Journal of Forecasting, 37 (2021) 388-427. https://doi.org/10.1016/j.ijforecast.2020.06.008
[13]. G. Van Houdt, C. Mosquera, G. Nápoles, A review on the long short-term memory model, Artif Intell Rev, 53 (2020) 5929–5955. https://doi.org/10.1007/s10462-020-09838-1
[2]. J. Zaheer, J. Jeon, S.B. LeeΙΙ, J.S. Kim, Effect of Particulate Matter on Human Health, Prevention, and Imaging Using PET or SPECT, Prog. Med. Phys., 29 (2018) 81-91. https://doi.org/10.14316/pmp.2018.29.3.81
[3]. Bộ TN&MT, Báo cáo Hiện trạng Môi trường Việt Nam giai đoạn 2016-2020, 2021.
[4]. Phạm Ngọc Đăng, Thực trạng chất lượng môi trường không khí Việt Nam giai đoạn 2016 – 2020, xác định các thách thức và đề xuất các giải pháp cải thiện trong 5 năm tới, Tạp chí Môi trường số tháng 1 (2022).
[5]. V. I. Kontopoulou, A. D. Athanasios, I. Kakkos, G. K. Matsopoulos., A Review of ARIMA vs. Machine Learning Approaches for Time Series Forecasting in Data Driven Networks, Future Internet, 15 (2023) 255. https://doi.org/10.3390/fi15080255
[6]. Peng Chen, Aichen Niu, Duanyang Liu, Wei Jiang, Bin Ma, Time Series Forecasting of Temperatures using SARIMA: An Example from Nanjing, IOP Conference Series: Materials Science and Engineering, 394 (2018) 052024. https://doi.org/10.1088/1757-899X/394/5/052024
[7]. Q. Song, B. S. Chissom, Forecasting enrollments with fuzzy time series – Part I. Fuzzy Sets and Systems, 54 (1993) 1–9. https://doi.org/10.1016/0165-0114(93)90355-L
[8]. C. R. Staudemeyer, E. R. Morris, Understanding LSTM -- a tutorial into Long Short-Term Memory Recurrent Neural Networks, (2019) 1-42, https://doi.org/10.48550/arXiv.1909.09586
[9]. Ahmed Tealab, Time series forecasting using artificial neural networks methodologies: A systematic review, Future Computing and Informatics Journal, 3 (2018) 334-340. https://doi.org/10.1016/j.fcij.2018.10.003
[10]. Gjylapi, Dezdemona & Proko, Eljona, Recurrent Neural Networks in Time Series Prediction, 2017.
[11]. David Kriesel, A Brief Introduction to Neural Networks, NeuralNetworks, 2007.
[12]. Hansika Hewamalage, Christoph Bergmeir, Kasun Bandara, Recurrent Neural Networks for Time Series Forecasting: Current status and future directions, International Journal of Forecasting, 37 (2021) 388-427. https://doi.org/10.1016/j.ijforecast.2020.06.008
[13]. G. Van Houdt, C. Mosquera, G. Nápoles, A review on the long short-term memory model, Artif Intell Rev, 53 (2020) 5929–5955. https://doi.org/10.1007/s10462-020-09838-1
Tải xuống
Chưa có dữ liệu thống kê
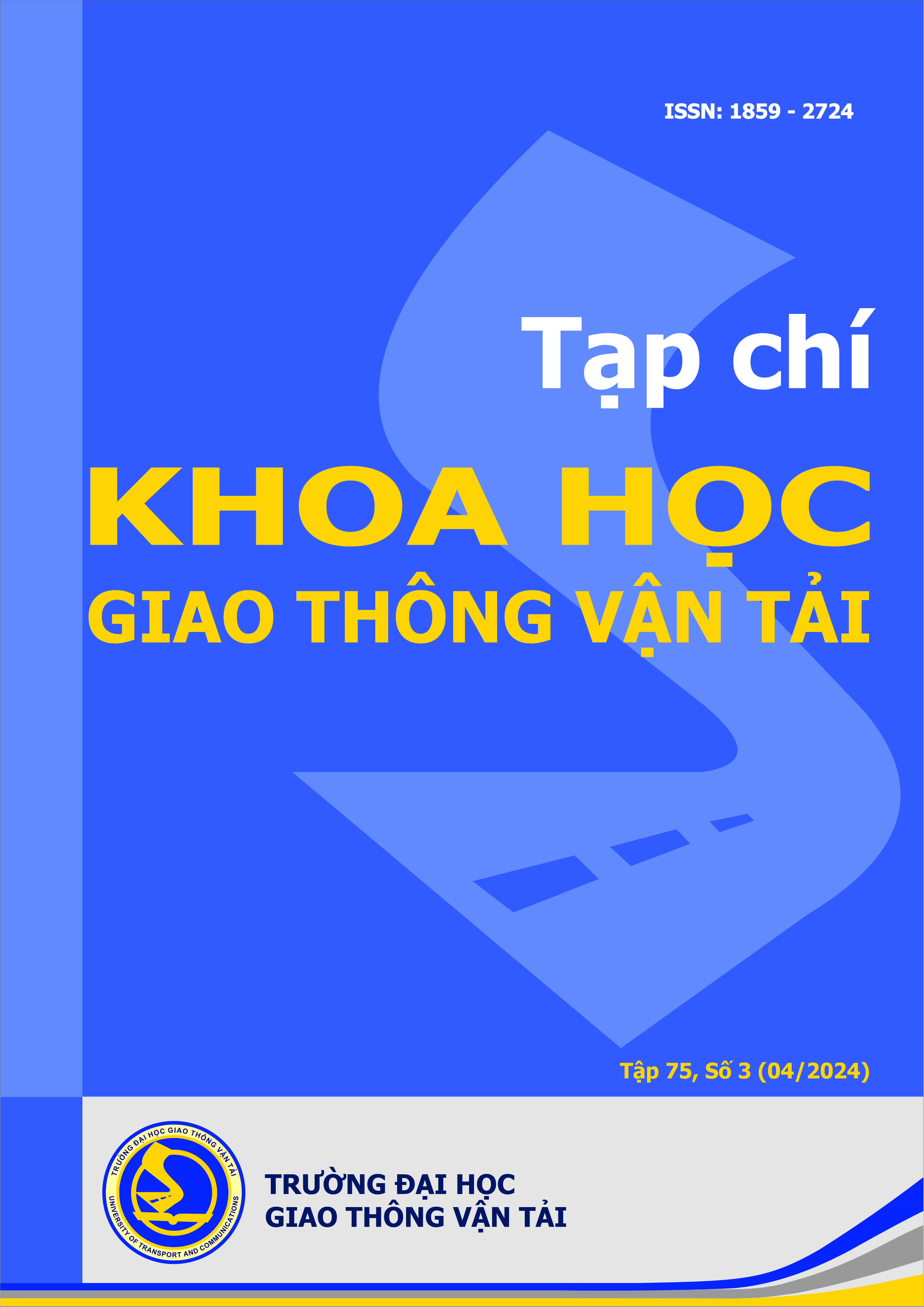
Nhận bài
18/12/2023
Nhận bài sửa
16/03/2024
Chấp nhận đăng
31/03/2024
Xuất bản
15/04/2024
Chuyên mục
Công trình khoa học
Kiểu trích dẫn
Cao Minh, Q., & Phạm Hồng, H. (1713114000). Dự báo ô nhiễm không khí từ dữ liệu quan trắc sử dụng các mô hình trí tuệ nhân tạo. Tạp Chí Khoa Học Giao Thông Vận Tải, 75(3), 1404-1412. https://doi.org/10.47869/tcsj.75.3.7
Số lần xem tóm tắt
2
Số lần xem bài báo
1