Một phương pháp nâng cao hiệu quả dự báo dữ liệu tuyển sinh dựa trên chuỗi thời gian mờ
Email:
phongpd@utc.edu.vn
Từ khóa:
dự báo, chuỗi thời gian mờ, giải mờ, quan hệ logic mờ.
Tóm tắt
Trong vài thập kỷ gần đây, nhiều nghiên cứu về mô hình dự báo dựa trên chuỗi thời gian mờ đã được đề xuất. Có nhiều nhân tố ảnh hưởng đến kết quả dự báo đã được nhiều tác giả tập trung nghiên cứu như kỹ thuật chia khoảng tập nền, các luật dự báo và kỹ thuật giải mờ. Tuy nhiên, các kết quả nghiên cứu vẫn còn nhiều hạn chế và chưa làm hài lòng người sử dụng. Trong bài báo này, chúng tôi đề xuất một phương pháp nâng cao hiệu quả của mô hình dự báo chuỗi thời gian mờ trên cơ sở kết hợp tối ưu các khoảng chia tập nền bằng thuật toán tối ưu bầy đàn và kỹ thuật giải mờ mới hiệu quả. Mô hình dự báo được đề xuất được ứng dụng để dự báo số sinh viên nhập học của Trường Đại học Alabama từ năm 1971 đến năm 1992. Kết quả thực nghiệm cho thấy, mô hình dự báo được đề xuất hiệu quả hơn các mô hình dự báo hiện có đối với cả chuỗi thời gian mờ bậc nhất và chuỗi thời gian mờ bậc cao.Tài liệu tham khảo
[1]. G. E. P. Box, G. Jenkins, Time Series Analysis, Forecasting and Control, Holden-Day, San Francisco, CA, 1970.
[2]. Q. Song, B.S. Chissom, Fuzzy Time Series and its Model, Fuzzy set and systems, 54 (1993) 269-277. https://doi.org/10.1016/0165-0114(93)90372-O
[3]. Q. Song, B.S. Chissom, Forecasting Enrollments with Fuzzy Time Series – Part I, Fuzzy set and systems, 54 (1993) 1-9. https://doi.org/10.1016/0165-0114(93)90355-L
[4]. Q. Song, B.S. Chissom, Forecasting Enrollments with Fuzzy Time Series – Part II, Fuzzy set and systems, 62 (1994) 1-8. https://doi.org/10.1016/0165-0114(94)90067-1
[5]. S. M. Chen, Forecasting Enrollments based on Fuzzy Time Series, Fuzzy set and systems, 81, (1996) 311-319. https://doi.org/10.1016/0165-0114(95)00220-0
[6]. S. M. Chen, Forecasting Enrollments based on high-order Fuzzy Time Series, Int. Journal: Cybernetic and Systems, 3 (2002) 1-16. https://doi.org/10.1080/019697202753306479
[7]. H. K. Yu, Weighted fuzzy time series models for TAIEX forecasting, Physica A: Statistical Mechanics and its Applications, 349 (2005) 609–624. https://doi.org/10.1016/j.physa.2004.11.006
[8]. S. M. Chen, N. Y. Chung, Forecasting enrolments of students by using fuzzy time series and genetic algorithms, International journal of information and management sciences, 17 (2006) 1–18.
[9]. S. M. Chen, N. Y. Chung, Forecasting enrollments using high-order fuzzy time series and genetic algorithms, International of Intelligent Systems, 21 (2006b) 485-501. https://doi.org/10.1002/int.20145
[10]. L. W. Lee, L. H. Wang, S. M. Chen, Temperature prediction and TAIFEX forecasting based on fuzzy logical relationships and genetic algorithms, Expert Systems with Applications, 33 (2007) 539-550. https://doi.org/10.1016/j.eswa.2006.05.015
[11]. L. W. Lee, L. H. Wang, S. M. Chen, Temperature prediction and TAIFEX forecasting based on high-order fuzzy logical relationships and genetic simulated annealing techniques, Expert Systems with Applications, 34 (2008) 328–336. https://doi.org/10.1016/j.eswa.2006.09.007
[12]. S. M. Chen, X. Y. Zou, G. C, Gunawan, Fuzzy time series forecasting based on proportions of intervals and particle swarm optimization techniques, Information Sciences, 500 (2019) 127–139.
https://doi.org/10.1016/j.ins.2019.05.047
[13]. I-H. Kuo, S.-J. Horng, T-W. Kao, T-L. Lin, C-L. Lee, Y. Pan, An improved method for forecasting enrolments based on fuzzy time series and particle swarm optimization, Expert systems with applications, 36 (2009) 6108–6117. https://doi.org/10.1016/j.eswa.2008.07.043
[14]. I-H. Kuo, S-J. Horng, Y-H. Chen, R-S. Run, T-W. Kao, R-J. Chen, J-L. Lai, T-L. Lin, “Forecasting TAIFEX based on fuzzy time series and particle swarm optimization”, Expert Systems with Applications, 37 (2010) 1494–1502. https://doi.org/10.1016/j.eswa.2009.06.102
[15]. Y. L. Huang, S. J. Horng, M. He, P. Fan, T. W. Kao, M. K. Khan, A hybrid forecasting model for enrollments based on aggregated fuzzy time series and particle swarm optimization, Expert Systems with Applications, 38 (2011) 8014 – 8023. https://doi.org/10.1007/s10489-016-0857-0
[16]. N. V. Tinh, N. C. Dieu, A New Hybrid Fuzzy Time Series Forecasting Model Combined the Time -Variant Fuzzy Logical Relationship Groups with Particle Swam Optimization, Computer Science and Engineering, 7 (2017) 52-66.
[17]. Nguyễn Công Điều, Nghiêm Văn Tính, Dự báo chuỗi thời gian mờ dựa trên nhóm quan hệ mờ phụ thuộc thời gian và tối ưu bầy đàn, Kỷ yếu Hội thảo quốc gia về nghiên cứu cơ bản và ứng dụng công nghệ thông tin Cần Thơ, 2016, 125-133.
[18]. N. Y Wang, S. M. Chen, Temperature prediction and TAIFEX forecasting based on automatic clustering techniques and two-factors high-order fuzzy time series, Expert Systems with Applications, 36 (2009) 2143-2154. https://doi.org/10.1016/j.eswa.2007.12.013
[19]. C. H. Cheng, G-W. Cheng, J-W. Wang, Multi-attribute fuzzy time series method based on fuzzy clustering, Expert Systems with Applications, 34 (2008) 1235–1242. https://doi.org/10.1016/j.eswa.2006.12.013
[20]. C. H. Wang, L. C. Hsu, Constructing and applying an improved fuzzy time series model: Taking the tourism industry for example, Expert Systems with Applications, 34 (2008) 2732-2738. https://doi.org/10.1016/j.eswa.2007.05.042
[21]. M. Bose, K. Mali, Designing fuzzy time series forecasting models: A survey, International Journal of Approximate Reasoning, 111 (2019) 78–99. https://doi.org/10.1016/j.ijar.2019.05.002
[22]. J. Kennedy, R. C. Eberhart, Particle Swarm Optimization, In Proceedings of the IEEE International Conference on Neural Networks, Piscataway, New Jersey. IEEE Service Center, 1995, 1942–1948.
[23]. R. C. Eberhart, J. Kennedy, A new optimizer using particle swarm theory, Proceedings of the Sixth International Symposium on Micro Machine and Human Science, Nagoya, Japan, 1995, 39-43. https://doi.org/10.1109/MHS.1995.494215
[24]. K. Huarng, Effective lengths of intervals to improve forecasting in fuzzy time series, Fuzzy Sets and Systems, 123 (2001b) 387-394. https://doi.org/10.1007/s10700-006-0025-9
[25]. V. R. Uslu, E. Bas, U. Yolcu, E. Egrioglu, A fuzzy time series approach based on weights determined by the number of recurrences of fuzzy relations, Swarm and Evolutionary Computation, 15 (2014) 19–26. https://doi.org/10.1016/j.swevo.2013.10.004
[2]. Q. Song, B.S. Chissom, Fuzzy Time Series and its Model, Fuzzy set and systems, 54 (1993) 269-277. https://doi.org/10.1016/0165-0114(93)90372-O
[3]. Q. Song, B.S. Chissom, Forecasting Enrollments with Fuzzy Time Series – Part I, Fuzzy set and systems, 54 (1993) 1-9. https://doi.org/10.1016/0165-0114(93)90355-L
[4]. Q. Song, B.S. Chissom, Forecasting Enrollments with Fuzzy Time Series – Part II, Fuzzy set and systems, 62 (1994) 1-8. https://doi.org/10.1016/0165-0114(94)90067-1
[5]. S. M. Chen, Forecasting Enrollments based on Fuzzy Time Series, Fuzzy set and systems, 81, (1996) 311-319. https://doi.org/10.1016/0165-0114(95)00220-0
[6]. S. M. Chen, Forecasting Enrollments based on high-order Fuzzy Time Series, Int. Journal: Cybernetic and Systems, 3 (2002) 1-16. https://doi.org/10.1080/019697202753306479
[7]. H. K. Yu, Weighted fuzzy time series models for TAIEX forecasting, Physica A: Statistical Mechanics and its Applications, 349 (2005) 609–624. https://doi.org/10.1016/j.physa.2004.11.006
[8]. S. M. Chen, N. Y. Chung, Forecasting enrolments of students by using fuzzy time series and genetic algorithms, International journal of information and management sciences, 17 (2006) 1–18.
[9]. S. M. Chen, N. Y. Chung, Forecasting enrollments using high-order fuzzy time series and genetic algorithms, International of Intelligent Systems, 21 (2006b) 485-501. https://doi.org/10.1002/int.20145
[10]. L. W. Lee, L. H. Wang, S. M. Chen, Temperature prediction and TAIFEX forecasting based on fuzzy logical relationships and genetic algorithms, Expert Systems with Applications, 33 (2007) 539-550. https://doi.org/10.1016/j.eswa.2006.05.015
[11]. L. W. Lee, L. H. Wang, S. M. Chen, Temperature prediction and TAIFEX forecasting based on high-order fuzzy logical relationships and genetic simulated annealing techniques, Expert Systems with Applications, 34 (2008) 328–336. https://doi.org/10.1016/j.eswa.2006.09.007
[12]. S. M. Chen, X. Y. Zou, G. C, Gunawan, Fuzzy time series forecasting based on proportions of intervals and particle swarm optimization techniques, Information Sciences, 500 (2019) 127–139.
https://doi.org/10.1016/j.ins.2019.05.047
[13]. I-H. Kuo, S.-J. Horng, T-W. Kao, T-L. Lin, C-L. Lee, Y. Pan, An improved method for forecasting enrolments based on fuzzy time series and particle swarm optimization, Expert systems with applications, 36 (2009) 6108–6117. https://doi.org/10.1016/j.eswa.2008.07.043
[14]. I-H. Kuo, S-J. Horng, Y-H. Chen, R-S. Run, T-W. Kao, R-J. Chen, J-L. Lai, T-L. Lin, “Forecasting TAIFEX based on fuzzy time series and particle swarm optimization”, Expert Systems with Applications, 37 (2010) 1494–1502. https://doi.org/10.1016/j.eswa.2009.06.102
[15]. Y. L. Huang, S. J. Horng, M. He, P. Fan, T. W. Kao, M. K. Khan, A hybrid forecasting model for enrollments based on aggregated fuzzy time series and particle swarm optimization, Expert Systems with Applications, 38 (2011) 8014 – 8023. https://doi.org/10.1007/s10489-016-0857-0
[16]. N. V. Tinh, N. C. Dieu, A New Hybrid Fuzzy Time Series Forecasting Model Combined the Time -Variant Fuzzy Logical Relationship Groups with Particle Swam Optimization, Computer Science and Engineering, 7 (2017) 52-66.
[17]. Nguyễn Công Điều, Nghiêm Văn Tính, Dự báo chuỗi thời gian mờ dựa trên nhóm quan hệ mờ phụ thuộc thời gian và tối ưu bầy đàn, Kỷ yếu Hội thảo quốc gia về nghiên cứu cơ bản và ứng dụng công nghệ thông tin Cần Thơ, 2016, 125-133.
[18]. N. Y Wang, S. M. Chen, Temperature prediction and TAIFEX forecasting based on automatic clustering techniques and two-factors high-order fuzzy time series, Expert Systems with Applications, 36 (2009) 2143-2154. https://doi.org/10.1016/j.eswa.2007.12.013
[19]. C. H. Cheng, G-W. Cheng, J-W. Wang, Multi-attribute fuzzy time series method based on fuzzy clustering, Expert Systems with Applications, 34 (2008) 1235–1242. https://doi.org/10.1016/j.eswa.2006.12.013
[20]. C. H. Wang, L. C. Hsu, Constructing and applying an improved fuzzy time series model: Taking the tourism industry for example, Expert Systems with Applications, 34 (2008) 2732-2738. https://doi.org/10.1016/j.eswa.2007.05.042
[21]. M. Bose, K. Mali, Designing fuzzy time series forecasting models: A survey, International Journal of Approximate Reasoning, 111 (2019) 78–99. https://doi.org/10.1016/j.ijar.2019.05.002
[22]. J. Kennedy, R. C. Eberhart, Particle Swarm Optimization, In Proceedings of the IEEE International Conference on Neural Networks, Piscataway, New Jersey. IEEE Service Center, 1995, 1942–1948.
[23]. R. C. Eberhart, J. Kennedy, A new optimizer using particle swarm theory, Proceedings of the Sixth International Symposium on Micro Machine and Human Science, Nagoya, Japan, 1995, 39-43. https://doi.org/10.1109/MHS.1995.494215
[24]. K. Huarng, Effective lengths of intervals to improve forecasting in fuzzy time series, Fuzzy Sets and Systems, 123 (2001b) 387-394. https://doi.org/10.1007/s10700-006-0025-9
[25]. V. R. Uslu, E. Bas, U. Yolcu, E. Egrioglu, A fuzzy time series approach based on weights determined by the number of recurrences of fuzzy relations, Swarm and Evolutionary Computation, 15 (2014) 19–26. https://doi.org/10.1016/j.swevo.2013.10.004
Tải xuống
Chưa có dữ liệu thống kê
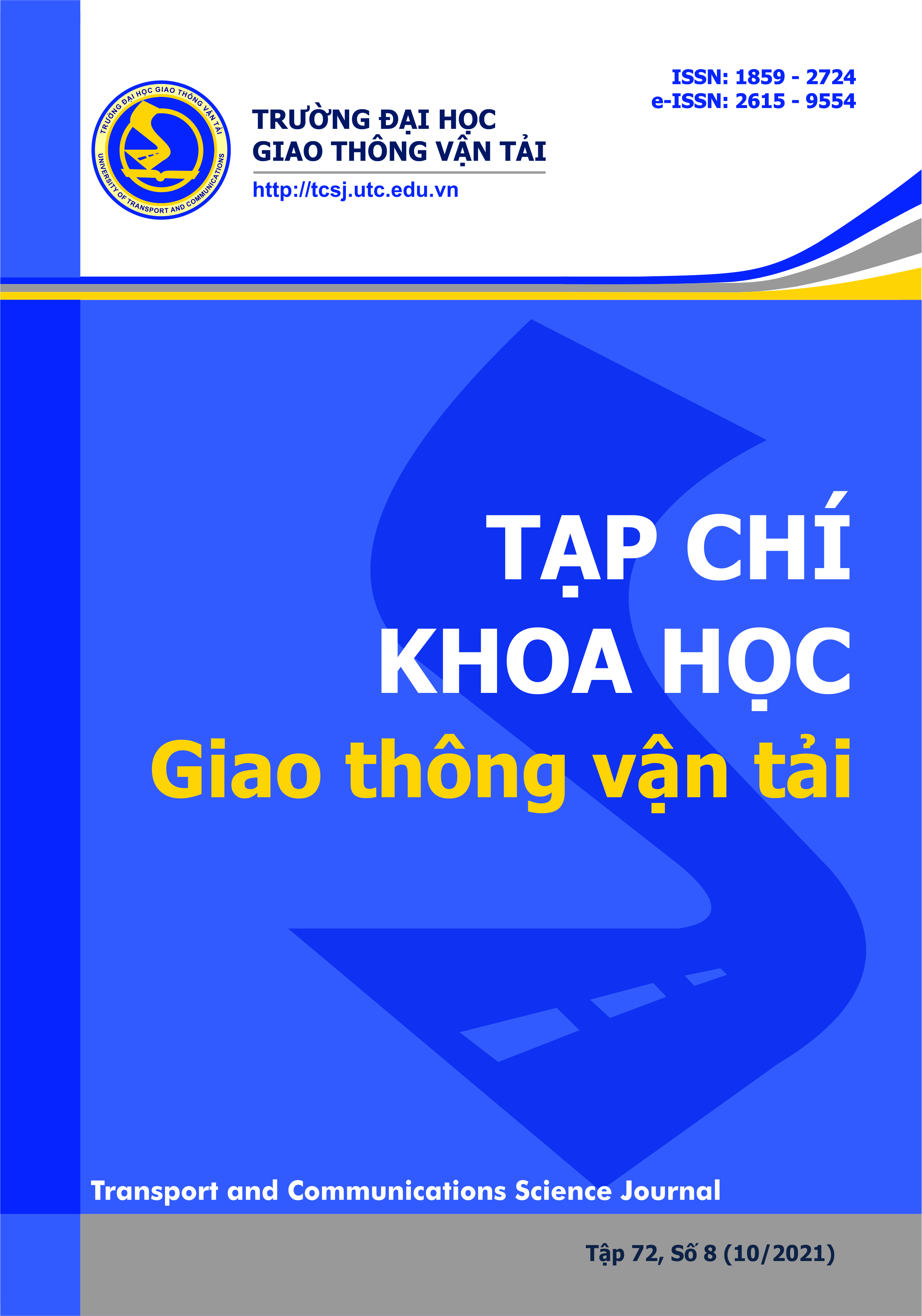
Nhận bài
15/06/2021
Nhận bài sửa
29/08/2021
Chấp nhận đăng
03/09/2021
Xuất bản
15/10/2021
Chuyên mục
Công trình khoa học
Kiểu trích dẫn
Nguyễn Văn, K., Nguyễn Đình, B., Nguyễn Bảo, T., & Phạm Đình, P. (1634230800). Một phương pháp nâng cao hiệu quả dự báo dữ liệu tuyển sinh dựa trên chuỗi thời gian mờ. Tạp Chí Khoa Học Giao Thông Vận Tải, 72(8), 967-981. https://doi.org/10.47869/tcsj.72.8.10
Số lần xem tóm tắt
350
Số lần xem bài báo
640